Meet Instinct- our 19 active channel EEG headset with powerful on-board signal processing capabilities and motorized electrodes for quick set-up.
Record. Analyze. Innovate on the go.

redefine
what
it means
to be human
We create non-invasive Brain-Computer Interface solutions for human brain to interact with technology.

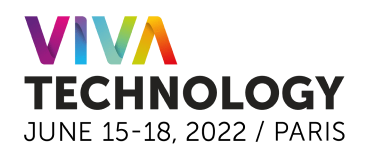
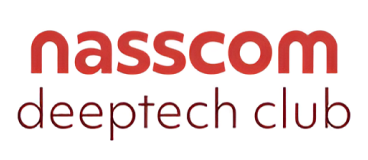

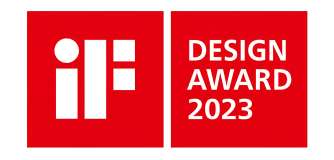
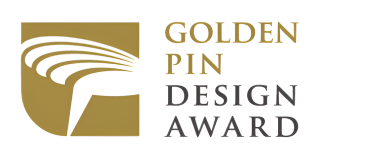
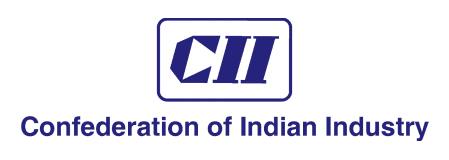
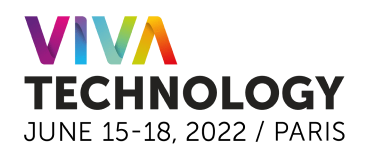
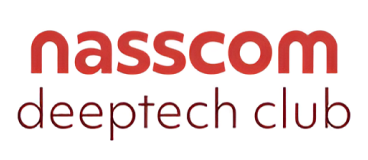

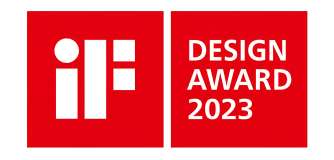
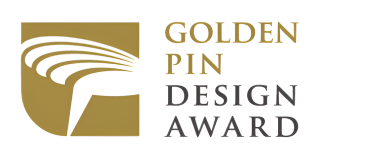
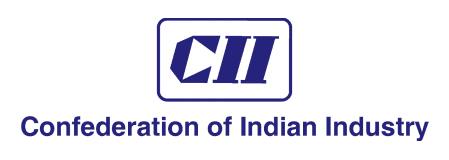
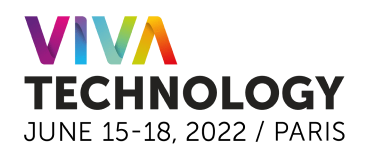
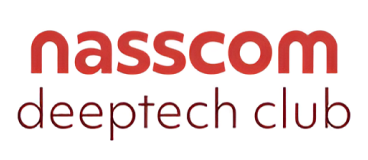

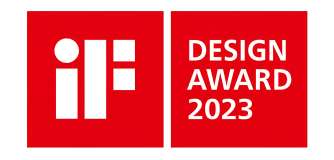
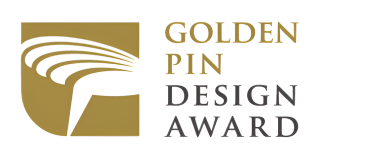
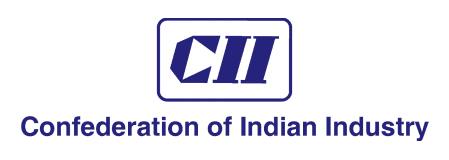
unlock mankind's true potential to foster it's evolution
Our technology captures brain signals, enabling humans to interact with tech devices using just their thoughts, pushing beyond their biological limits.

Nexstem's BCI technology ecosystem enables you to record, visualize and analyze EEG data to develop your unique use case.
Our end-to-end solutions include our BCI headset- Instinct which runs a custom developed OS for powerful bio-signal processing and analysis. Pre-bundled apps on the OS let you get started with sophisticated BCI functionalities right out of the box.

Innovation is at core of Nexstem's DNA. All technologies have been developed from ground up, re-thinking and re-designing the foundations to make BCI more accessible. We actively pursue research to continuously push the possibilities of neuroscience & engineering.

We are a team of innovators and dreamers working to create a world-class technology space with our user-friendly and accessible solutions for researchers, developers, and consumers. Our goal is to make brain-controlled technology a reality for everyone.




a new
gateway to
the brain
brain
implement powerful BCI workflows
Collect, decode, and demystify various bio-signals to gain sophisticated insights on the workings of the human brain.


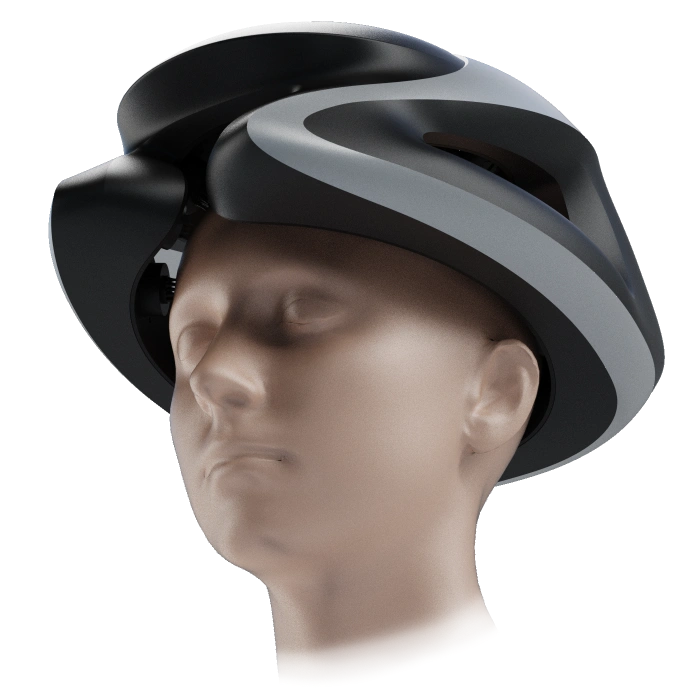
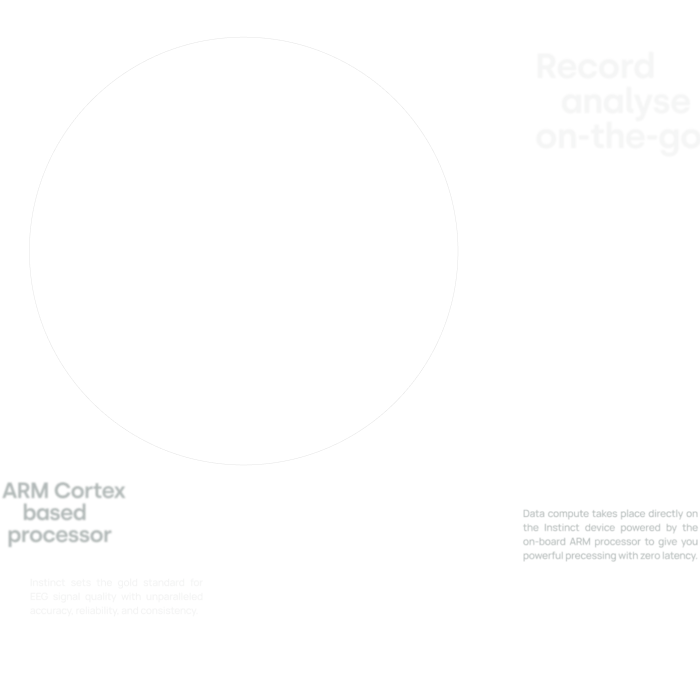

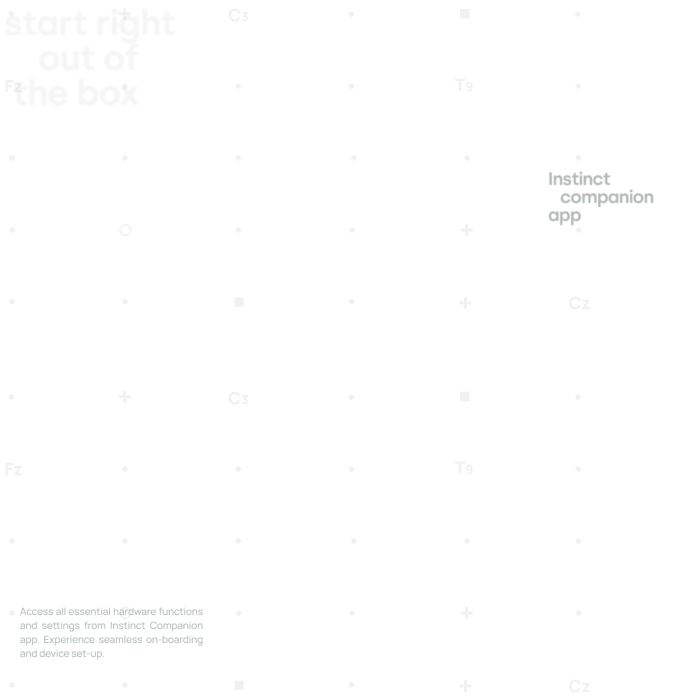


best-in-class signal acquisition
19 active channel auto-adjusting electrodes covers every head shape and size with set-up time <5 mins without compromising high quality EEG signals.
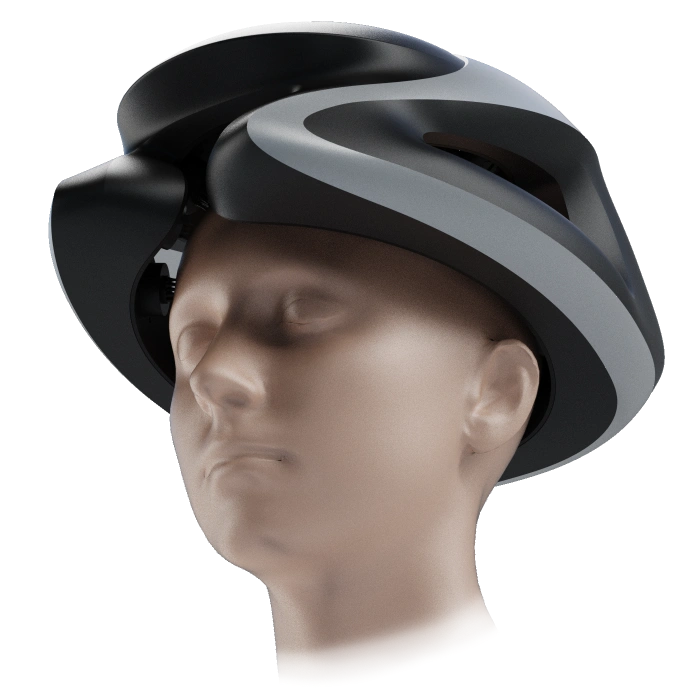
record, analyse innovate on-the-go
Data compute takes place directly on the Instinct device powered by the on-board ARM processor to give you powerful processing with zero latency.

start right out of the box
Access all essential hardware functions and settings from Instinct Companion app. Experience seamless on-boarding and device set-up.
.webp)
21 auto-adjusting electrodes cover every possible use with no compromise on the bio-signal quality.

Get your hands on the Instinct headset today at a special introductory price for limited time period. Enjoy access to Instinct Apps, SDK and APIs and entire ecosystem for free.
*Price exclusive shipping & taxes. Offer is valid for limited time period.
.webp)
push the boundaries of possible
BCI is at the forefront of neuroscience research and neuro-rehabilitation activities adding a new dimension to bio-signal driven healthcare. Direct brain control of prosthetic limbs is reimagining the quality of life limit for individuals with limb loss

Integration of neural data from BCI headsets with personal health tracking and lifestyle management devices is paving the way for more intuitive and responsive wearable devices.
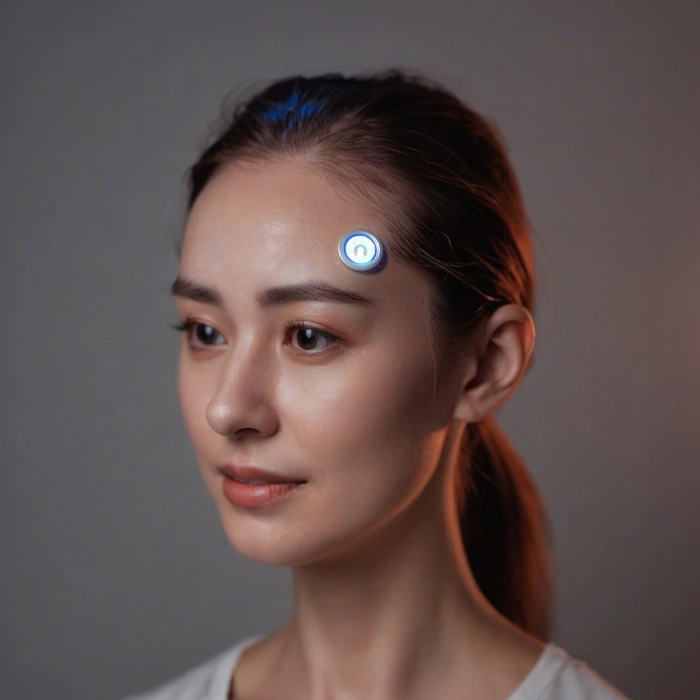
BCI is transforming the gaming and e-sports industries by creating immersive, mind-controlled gaming experiences, elevating player interaction and engagement to unprecedented levels.

Real-time neurofeedback for performance optimisation and injury prevention, offers neo-athletes a cutting-edge over competitors. BCI is enabling athletes unlock peak sports performance every time.
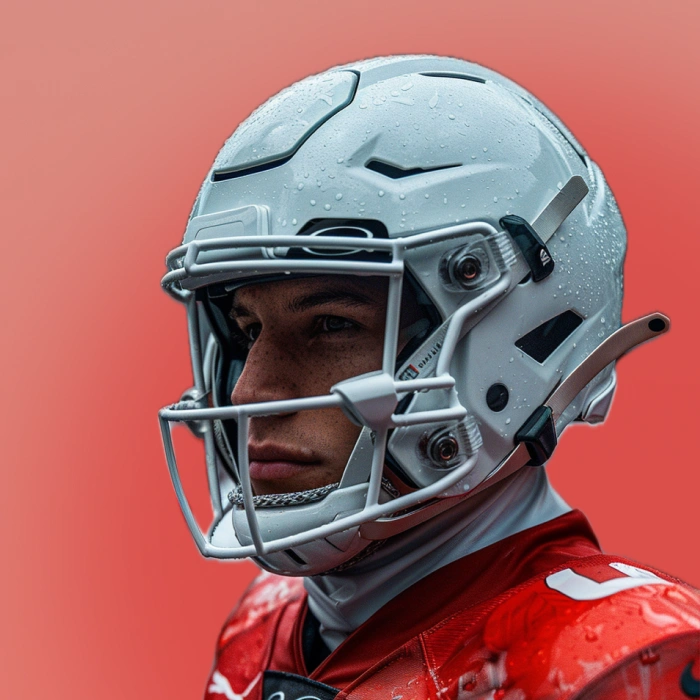

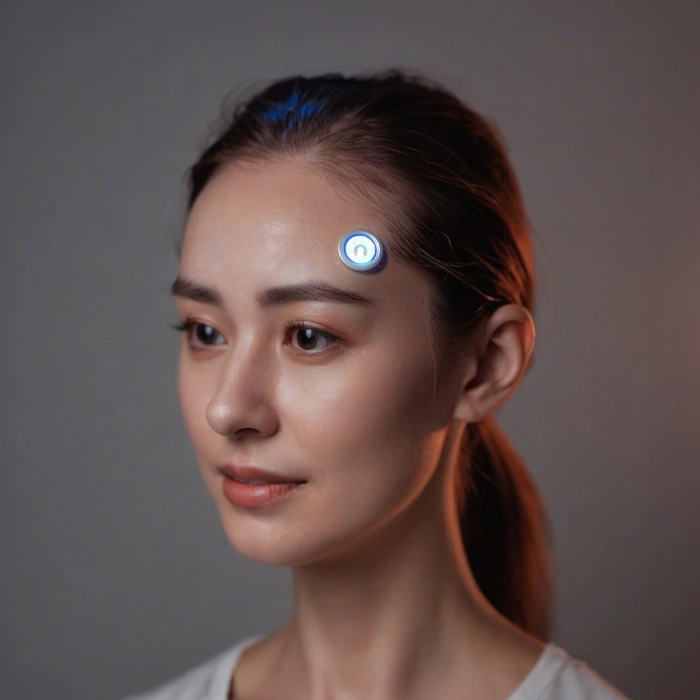

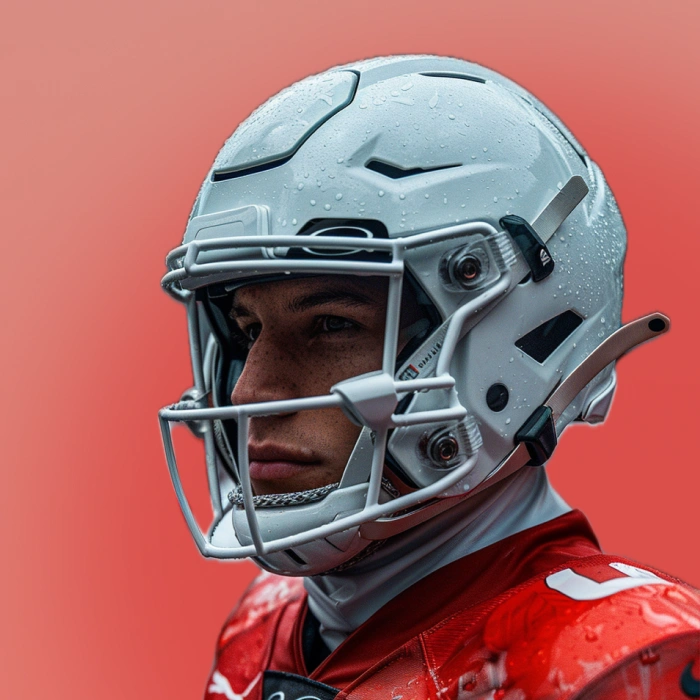

.webp)
.webp)
powerful tools for
‘sense-making’
Nexstem Apps
Leverage powerful hardware functionality and data processing algorithms right out of the box.

Instinct OS
Custom-built OS to complement the Instinct headset for on-device seamless bio-signal processing.

Developer Tools
InstinctSDK and InstinctAPIs give you first hand access to the Instinct hardware.

what Nexstem has been up to lately
get the latest pulse in the bci domain
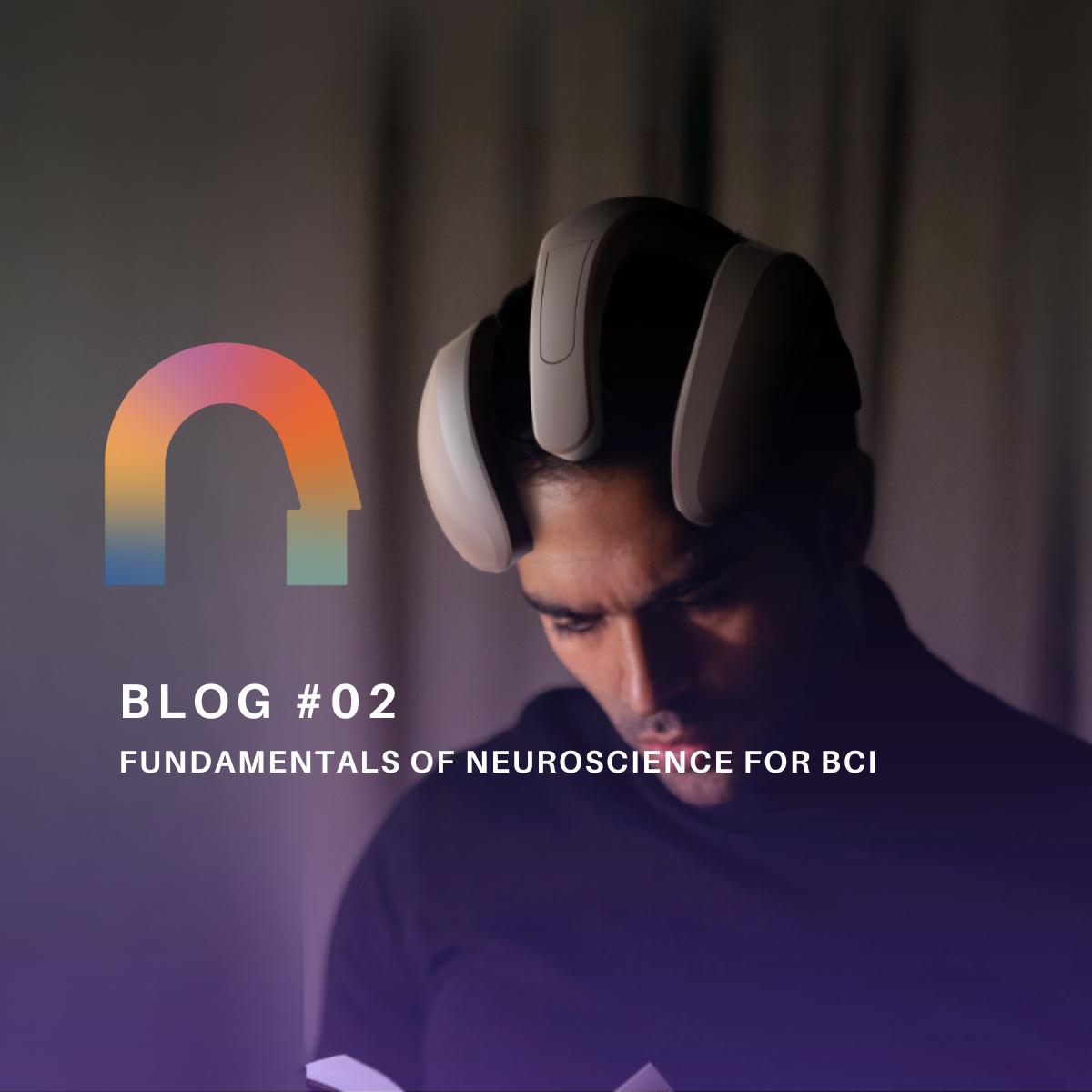
Welcome back to our BCI crash course! In the previous blog, we explored the basics of BCIs and different approaches to decoding brain signals. Today, we will explore the fascinating world of neuroscience to understand the foundation upon which these incredible technologies are built. This blog will focus on the electrical activity of the brain, particularly relevant for EEG-based BCIs. By understanding how neurons communicate and generate the rhythmic oscillations that EEG measures, we can gain valuable insights into the development and application of BCI systems.
Basic Brain Anatomy and Function: Your Brain's Control Center
The brain, the most complex organ in the human body, is the command center for our thoughts, emotions, and actions. To understand how BCIs tap into this intricate network, let's explore some key anatomical structures and their functions.
Brain Divisions: A Three-Part Harmony
The brain is broadly divided into three main sections:
- Forebrain: The largest and most evolved part of the brain, the forebrain is responsible for higher-level cognitive functions like language, reasoning, and problem-solving. It also processes sensory information from our environment, controls voluntary movement, and regulates emotions and motivations.
- Midbrain: Situated between the forebrain and hindbrain, the midbrain plays a crucial role in relaying sensory information to higher brain centers. It's also involved in motor control, particularly for eye movements, and in regulating sleep-wake cycles and arousal.
- Hindbrain: The oldest and most primitive part of the brain, the hindbrain is responsible for controlling vital autonomic functions such as breathing, heart rate, and blood pressure. It also coordinates balance and movement.

For BCI applications, the forebrain, particularly the cerebrum, is of primary interest. This is where conscious thought, decision-making, and voluntary actions originate.
Cerebral Cortex: The Brain's Outer Layer
The cerebrum's outer layer, the cerebral cortex, is a wrinkled sheet of neural tissue responsible for many of our higher cognitive abilities. It's divided into four lobes, each with specialized functions:
- Frontal Lobe: The "executive center" of the brain, the frontal lobe is responsible for planning, decision-making, working memory, and voluntary movement. It plays a crucial role in higher-level cognitive functions like reasoning, problem-solving, and language production. Damage to the frontal lobe can impair these functions and lead to changes in personality and behavior.
- Parietal Lobe: The parietal lobe processes sensory information related to touch, temperature, pain, and spatial awareness. It also integrates sensory input from different modalities, helping us form a coherent perception of our surroundings. Damage to the parietal lobe can cause difficulties with spatial navigation, object recognition, and body awareness.
- Temporal Lobe: The temporal lobe is involved in auditory processing, language comprehension, and memory formation. It contains structures like the hippocampus, crucial for long-term memory, and the amygdala, involved in processing emotions, particularly fear and aggression. Damage to the temporal lobe can impair memory, language comprehension, and emotional processing.
- Occipital Lobe: Located at the back of the brain, the occipital lobe is dedicated to visual processing. It receives input from the eyes and interprets visual information, allowing us to perceive shapes, colors, and motion. Damage to the occipital lobe can lead to visual impairments, including blindness or difficulty recognizing objects.

Gray and White Matter: The Brain's Building Blocks
The brain is composed of two main types of tissue:
- Gray Matter: Gray matter gets its color from the densely packed cell bodies of neurons. It is primarily involved in processing information, making decisions, and controlling actions. Gray matter is found in the cerebral cortex, basal ganglia, thalamus, and other brain regions involved in higher-level cognitive functions.
- White Matter: White matter is composed of myelinated axons, the long, slender projections of neurons that transmit electrical signals. Myelin, a fatty substance, acts as an insulator, allowing signals to travel faster and more efficiently. White matter forms the "wiring" that connects different brain regions, enabling communication and coordination between them.
Neural Signaling and Brain Rhythms: The Electrical Symphony of Your Brain
To understand how EEG-based BCIs work, we need to dive deeper into how neurons communicate and generate the electrical signals that EEG measures. This intricate process involves a complex interplay of electrical impulses, chemical messengers, and rhythmic oscillations.
Neurons and Synapses: The Building Blocks of Communication
Neurons are specialized cells that transmit information throughout the nervous system. They have a unique structure:
- Dendrites: Branch-like extensions that receive signals from other neurons.
- Cell Body (Soma): Contains the nucleus and other cellular machinery.
- Axon: A long, slender fiber that transmits electrical signals away from the cell body.
- Synapse: A small gap between the axon of one neuron and the dendrite of another, where communication occurs.
Electrical Signaling: The Language of Neurons
Neurons communicate using electrical impulses called action potentials. These brief, rapid changes in electrical charge travel down the axon, triggered by a complex interplay of ion channels that regulate the flow of charged particles across the neuron's membrane.
Think of an action potential like a wave traveling down a rope. It's an all-or-nothing event; once triggered, it propagates down the axon at a constant speed and amplitude.
When an action potential reaches the synapse, it triggers the release of neurotransmitters, chemical messengers that cross the synaptic gap and bind to receptors on the receiving neuron. This binding can either excite or inhibit the receiving neuron, modulating its likelihood of firing its own action potential.
Neurotransmitters and Receptors: Fine-Tuning the Signals
Neurotransmitters are the brain's chemical messengers, playing a crucial role in regulating mood, cognition, and behavior. Here are some key neurotransmitters relevant to BCI applications:
- Glutamate: The primary excitatory neurotransmitter in the brain, involved in learning, memory, and synaptic plasticity.
- GABA (Gamma-Aminobutyric Acid): The primary inhibitory neurotransmitter, important for calming neural activity and preventing overexcitation.
- Dopamine: Involved in reward, motivation, and motor control, playing a crucial role in Parkinson's disease.
- Acetylcholine: Plays a vital role in muscle contraction, memory, and attention.
Each neurotransmitter binds to specific receptors on the receiving neuron, triggering a cascade of intracellular events that ultimately modulate the neuron's electrical activity.
EEG Rhythms and Oscillations: Decoding the Brain's Rhythms
EEG measures the synchronized electrical activity of large groups of neurons firing together, generating rhythmic oscillations that reflect different brain states. These oscillations are categorized into frequency bands:
- Delta (1-4 Hz): The slowest brainwaves, dominant during deep sleep and associated with memory consolidation.
- Theta (4-8 Hz): Prominent during drowsiness, meditation, and creative states, often linked to cognitive processing and working memory.
- Alpha (8-12 Hz): Associated with relaxed wakefulness, particularly with eyes closed. Alpha waves are suppressed during mental exertion and visual processing.
- Beta (12-30 Hz): Reflect active thinking, focus, and alertness. Increased beta activity is observed during tasks requiring sustained attention and cognitive effort.
- Gamma (30-100 Hz): The fastest brainwaves, associated with higher cognitive functions, sensory binding, and conscious awareness.
By analyzing these rhythmic patterns, EEG-based BCIs can decode user intent, mental states, and even diagnose neurological conditions.
Electroencephalography (EEG) and its Significance in BCI: Capturing the Brain's Electrical Whispers
EEG, as we've mentioned throughout this post, is a powerful tool for capturing the electrical activity of the brain, making it a cornerstone of many BCI systems. Let's explore how EEG works and why it's so valuable for decoding brain signals.
How EEG Works: Recording the Brain's Electrical Symphony
EEG measures the electrical potentials generated by synchronized neuronal activity in the cerebral cortex. This is achieved using electrodes placed on the scalp, which detect the tiny voltage fluctuations produced by these electrical currents.
The electrodes are typically arranged according to the 10-20 system, a standardized placement system that ensures consistent and comparable recordings across different individuals and research studies.
The 10-20 System: A Standardized Map for EEG Recording
The 10-20 system is the internationally standardized method for placing EEG electrodes. It provides a consistent framework for recording and interpreting EEG data, allowing researchers and clinicians worldwide to communicate and compare results effectively.
The system is based on specific anatomical landmarks on the skull:
- Nasion: The indentation at the top of the nose, between the eyebrows.
- Inion: The bony prominence at the back of the head.
- Preauricular Points: The depressions just in front of each ear.
Electrodes are placed at intervals of 10% or 20% of the total distance between these reference points, forming a grid-like pattern that covers the scalp.
Each electrode is labeled with a letter and a number:
- Letters: Represent the underlying brain region (Fp for prefrontal, F for frontal, C for central, P for parietal, T for temporal, O for occipital).
- Numbers: Indicate the hemisphere (odd numbers for the left, even numbers for the right, and z for midline).
This standardized system ensures that electrodes are consistently placed in the same locations across different individuals, facilitating reliable comparisons and analysis of EEG data.
High-Density vs. Low-Density Systems: A Matter of Resolution
EEG systems vary in the number of electrodes they use, ranging from a few electrodes in consumer-grade headsets to hundreds of electrodes in research-grade systems.
- High-Density Systems: Provide higher spatial resolution, allowing for more precise localization of brain activity. They are commonly used in research settings for investigating complex cognitive processes and mapping brain function.
- Low-Density Systems: Offer portability and affordability, making them suitable for consumer applications like neurofeedback, meditation training, and sleep monitoring. However, their lower spatial resolution limits their ability to pinpoint specific brain regions.
The choice of system depends on the specific application and the desired level of detail in capturing brain activity.
Types of EEG Electrodes: From Wet to Dry
Various types of EEG electrodes are available, each with its own advantages and disadvantages:
- Wet Electrodes: Require a conductive gel or paste to enhance electrical contact with the scalp. They generally provide better signal quality but can be more time-consuming to apply.
- Dry Electrodes: Don't require conductive gel, making them more convenient and user-friendly, but they might have slightly lower signal quality.
EEG Montages: Choosing Your Viewpoint
EEG montages refer to the way electrode pairs are connected to create the electrical signals displayed. Different montages highlight different aspects of brain activity and can influence the interpretation of EEG data.
Common montages include:
- Bipolar Montage: Each channel represents the voltage difference between two adjacent electrodes, emphasizing localized activity and minimizing the influence of distant sources.
- Referential Montage: Each channel represents the voltage difference between an active electrode and a common reference electrode (e.g., linked mastoids, average reference). This montage provides a broader view of brain activity across regions but can be more susceptible to artifacts from the reference electrode.
The choice of montage depends on the research question or BCI application. Bipolar montages are often preferred for studying localized brain activity, while referential montages are useful for examining activity across broader brain regions.
Further Reading and Resources:
- Principles of Neural Science – Kandel et al
- HarvardX: Fundamentals of Neuroscience, Part 1: The Electrical Properties of the Neuron (https://www.edx.org/learn/neuroscience/harvard-university-fundamentals-of-neuroscience-part-1-the-electrical-properties-of-the-neuron)
- HarvardX: Fundamentals of Neuroscience, Part 2: Neurons and Networks (https://www.edx.org/learn/neuroscience/harvard-university-fundamentals-of-neuroscience-part-2-neurons-and-networks)
- HarvardX: Fundamentals of Neuroscience, Part 3: The Brain (https://www.edx.org/learn/neuroscience/harvard-university-fundamentals-of-neuroscience-part-3-the-brain)
Ready to Dive Deeper into EEG Signal Processing?
This concludes our exploration of the fundamentals of neuroscience for BCI. In the next post, we'll dive into the practical aspects of EEG signal acquisition and processing, exploring the techniques used to extract meaningful information from the raw EEG data.
Stay tuned for our next BCI adventure!
.png)
Welcome to the first installment of our crash course on Brain-Computer Interfaces (BCIs)! This fascinating field holds immense potential for revolutionizing how we interact with technology and the world around us. In this series, we'll delve into the core concepts, methodologies, and applications of BCIs, providing you with a solid foundation to understand this exciting domain.
What is a BCI?
Brain-Computer Interfaces, or BCIs, are systems that establish a direct communication pathway between the brain and external devices. They bypass traditional neuromuscular pathways, allowing individuals to control machines or receive sensory feedback using only their brain activity. This groundbreaking technology has far-reaching implications for various fields, including healthcare, assistive technology, gaming, and even art.
At its core, a BCI system operates through three fundamental steps:
- Brain Signal Acquisition: Neural activity is recorded using various techniques, such as electroencephalography (EEG), electrocorticography (ECoG), or invasive electrode implants.
- Signal Processing: The acquired brain signals are processed and analyzed to extract meaningful patterns and features related to the user's intent or mental state.
- Output and Control: The extracted information is translated into commands that control external devices, such as prosthetic limbs, wheelchairs, or computer cursors.
BCIs offer a powerful means of communication and control, particularly for individuals with severe motor impairments or those suffering from locked-in syndrome. They open up a world of possibilities for restoring lost function, enhancing human capabilities, and even creating entirely new forms of human-computer interaction.
The Evolution of BCI over time
The history of brain-computer interfaces (BCIs) dates back to 1924 when Hans Berger first recorded human brain activity using electroencephalography (EEG). However, significant BCI research gained momentum in the 1970s at the University of California, Los Angeles (UCLA), focusing on using EEG signals for basic device control. Since then, advancements in the field have propelled BCI technology forward, including:
- Improved Electrodes and Sensors: Development of high-density electrode arrays and more sensitive sensors for better signal acquisition.
- Advanced Signal Processing Techniques: Sophisticated algorithms for filtering noise, artifact removal, and feature extraction from brain signals.
- Machine Learning Revolution: Application of machine learning algorithms for pattern recognition and classification, enabling more accurate decoding of user intent.
These advancements have led to impressive applications of BCIs:
- Restoring Lost Function: Controlling prosthetic limbs, wheelchairs, and communication devices for individuals with paralysis or locked-in syndrome.
- Assistive Technology: Developing tools for environmental control, such as controlling lights or appliances with brain signals.
- Gaming and Entertainment: Creating immersive and interactive experiences using brain-controlled interfaces.
- Neurofeedback and Therapy: Utilizing BCIs for treating conditions like ADHD, anxiety, and chronic pain.
- Cognitive Enhancement: Exploring the potential of BCIs for improving memory, attention, and other cognitive functions.
The future of BCIs is brimming with possibilities. As research progresses, we can expect even more groundbreaking applications that will further transform how we interact with the world and push the boundaries of human potential.
A Look at Different BCI Types
BCI systems are categorized based on their level of invasiveness, each with its own trade-offs in terms of signal quality, complexity, and risk. The three main types are:
Invasive BCI: Direct Access to the Source
Invasive BCIs involve surgically implanting electrodes directly into the brain tissue. This method offers the highest signal quality and allows for the most precise control. However, it also carries the highest risk of medical complications and requires significant expertise for implantation and maintenance.
Example: The Utah Array, a microelectrode array, is a prominent example of an invasive BCI used in research and clinical trials.
Applications: Primarily used for restoring lost motor function in individuals with paralysis or locked-in syndrome, enabling them to control prosthetic limbs, wheelchairs, and communication devices.
Semi-Invasive BCI (ECoG): Bridging the Gap
Semi-invasive BCIs, specifically Electrocorticography (ECoG), involve placing electrodes on the surface of the brain, beneath the skull. This approach offers a balance between signal quality and invasiveness, providing higher resolution than non-invasive methods while minimizing the risks associated with penetrating the brain tissue.
One notable company in this field is Synchron, which employs a minimally invasive endovascular procedure—similar to stent placement—to avoid open brain surgery. Their innovative approach allows for the safe and effective placement of electrodes, enabling patients to interact with technology using their thoughts. In a recent clinical trial, their first participant successfully connected with his wife by digitally controlling his computer through thought.
Applications: ECoG-based BCIs, including those developed by Synchron, are currently used in research settings for investigating brain function and exploring potential applications in epilepsy treatment and motor rehabilitation.
Non-Invasive BCIs: Exploring the Brain from the Outside
Non-invasive BCIs are the most common and accessible type, as they rely on external sensors to record brain activity without the need for surgery. These methods are generally safer and more comfortable for the user. However, the signal quality can be affected by noise and artifacts, requiring sophisticated signal processing techniques for accurate interpretation.
Examples:
- Electroencephalography (EEG): Measures electrical activity in the brain using electrodes placed on the scalp.
- Magnetoencephalography (MEG): Detects magnetic fields generated by brain activity.
- Functional Magnetic Resonance Imaging (fMRI): Measures brain activity by detecting changes in blood flow.
- Functional Near-Infrared Spectroscopy (fNIRS): Measures brain activity by monitoring changes in blood oxygenation
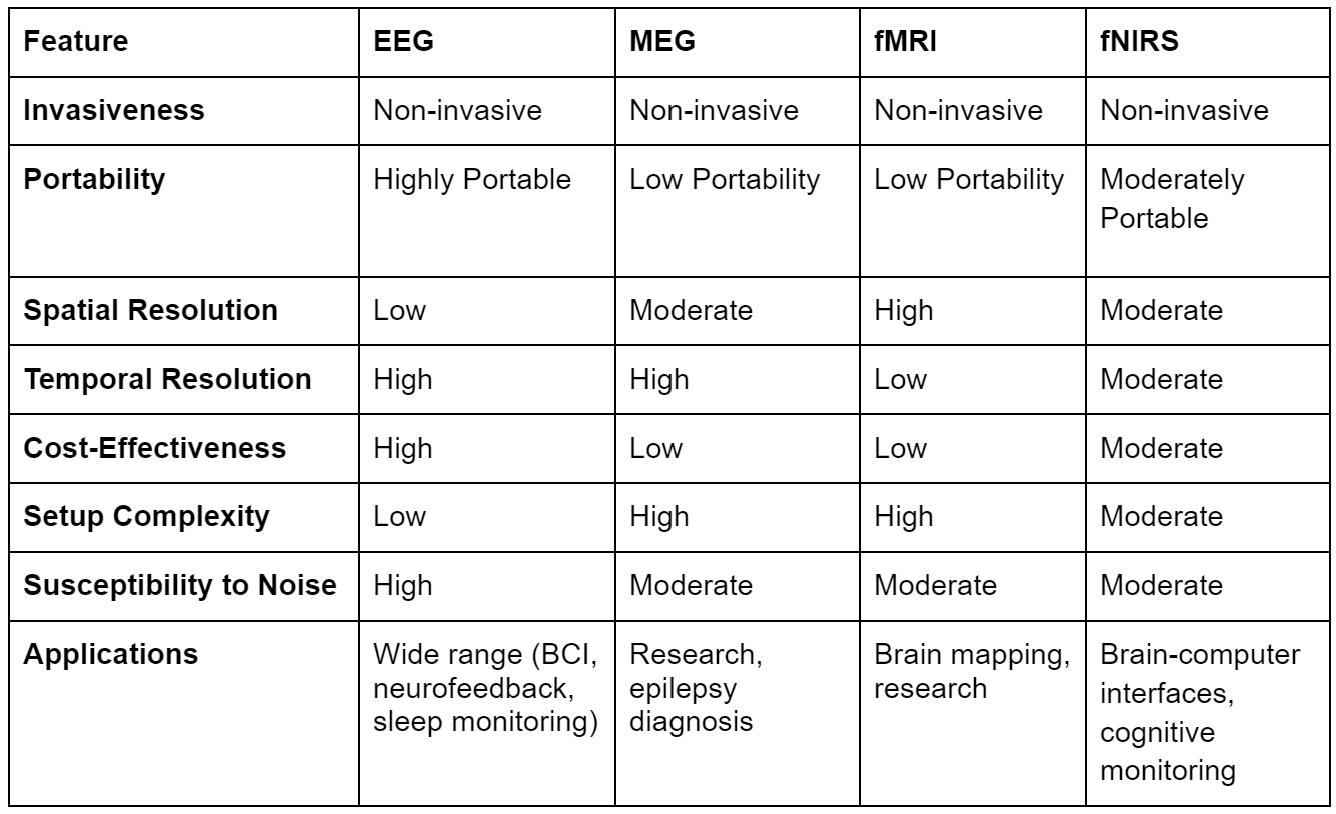
EEG-Based BCIs: A Deep Dive
Among the various non-invasive BCI approaches, electroencephalography (EEG) stands out as a particularly promising and widely adopted technology. EEG-based BCIs leverage the electrical activity generated by the brain, recorded through electrodes placed on the scalp. This method offers several key advantages:
High Temporal Resolution: EEG boasts excellent temporal resolution, capturing brain activity changes in milliseconds. This rapid sampling rate allows for real-time detection of subtle shifts in brain states, crucial for accurate and responsive BCI control.
Portability and Ease of Use: EEG systems are relatively portable and easy to set up, making them suitable for a variety of environments, from research labs to home settings. The non-invasive nature of EEG also contributes to its ease of use, as it doesn't require surgery or complex procedures.
Cost-Effectiveness: Compared to other neuroimaging techniques like fMRI or MEG, EEG is significantly more affordable. This accessibility makes it an attractive option for research, development, and widespread adoption of BCI technology.
Wide Range of Applications: EEG-based BCIs have demonstrated their versatility in a multitude of applications, including:
- Motor Imagery BCIs: Allow users to control devices by imagining specific movements.
- P300 Spellers: Enable users to spell words by focusing their attention on specific letters.
- Steady-State Visual Evoked Potential (SSVEP) BCIs: Utilize visual stimuli to elicit brain responses for control.
- Neurofeedback and Therapy: Provide real-time feedback on brain activity to help users learn to self-regulate their brain states for therapeutic purposes.
Addressing the Limitations:
The primary challenge lies in EEG’s lower spatial resolution compared to invasive or semi-invasive techniques. The electrical signals recorded by EEG electrodes are a mixture of activity from various brain regions, making it more difficult to pinpoint the precise source of the signal. However, advancements in signal processing and machine learning algorithms are continually improving the ability to extract meaningful information from EEG data, mitigating this limitation.
On the whole, EEG-based BCIs, especially when combined with fNIRS, offer a compelling mix of high temporal resolution, portability, affordability, and versatility. This combination enhances the ability to capture both electrical activity and hemodynamic responses in the brain, providing a more comprehensive understanding of brain function. These advantages have propelled EEG to the forefront of BCI research and development, driving innovation and expanding the potential applications of this transformative technology.
The Future is Brain-Powered
Brain-computer interfaces have emerged as a revolutionary technology with the potential to fundamentally change how we interact with the world around us. While various approaches exist, EEG-based BCIs stand out due to their unique combination of high temporal resolution, portability, cost-effectiveness, and versatility. From restoring lost motor function to enhancing cognitive abilities, the applications of EEG-based BCIs are vast and rapidly expanding.
As research and development continue to advance, we can expect even more groundbreaking innovations in the field of BCIs, leading to a future where our brains can seamlessly interact with technology, unlocking new possibilities for communication, control, and human potential.
Ready to Dive Deeper?
This concludes the first part of our crash course on Brain-Computer Interfaces. We've explored the fundamental concepts, different types of BCIs, and the advantages of EEG-based systems.
In the next installments of this series, we'll concern ourselves with specific aspects of BCI technology, covering topics such as:
- Fundamentals of Neuroscience for BCI: Understanding the brain's electrical activity and how it relates to BCI control.
- EEG Signal Acquisition and Processing: Exploring the techniques used to record and analyze EEG data.
- BCI Paradigms and Applications: Examining different types of BCI systems and their specific applications.
- Building Your Own BCI with Python: A hands-on guide to developing your own BCI applications.
Stay tuned for the next exciting chapter in our BCI journey!
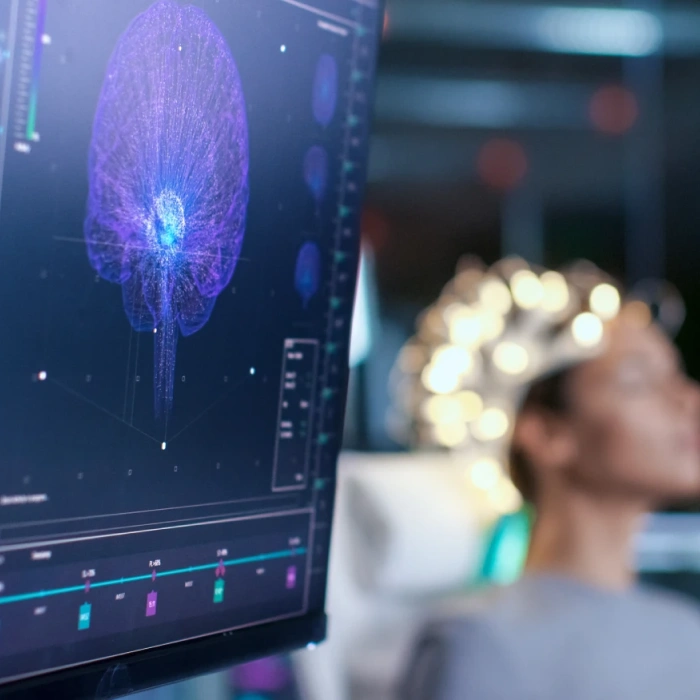
Discover how EEG's real-world applications are revolutionizing neuroscience and paving the way for new discoveries from clinical diagnostics to cognitive enhancement.
In the exciting world of neuroscience, researchers are on a mission to unravel the mysteries of the human brain. Electroencephalography (EEG) is an excellent tool, offering researchers an inside look into the intricate performance of electrical signals within the brain. In this exploration, we dive into the practical applications of EEG, shining a light on its importance and promising potential for researchers in the field.
What is an EEG Signal?
An EEG (Electroencephalogram) signal is a recording of the electrical activity generated by the neurons (nerve cells) in the brain. Neurons communicate with each other through electrical impulses, and these electrical signals can be detected and measured using electrodes placed on the scalp. The EEG signal reflects the synchronized activity of a large number of neurons firing in the brain.
The EEG signal is typically composed of different frequency components, known as brainwaves, which are classified into several bands:
- Delta (0.5-4 Hz): Associated with deep sleep and certain pathological conditions.
- Theta (4-8 Hz): Predominant in drowsiness and light sleep.
- Alpha (8-13 Hz): Dominant in relaxed wakefulness, often seen with closed eyes.
- Beta (13-30 Hz): Associated with active, alert, and focused mental states.
- Gamma (30-40 Hz and above): Linked to higher cognitive functions, perception, and consciousness.
Monitoring and analyzing EEG signals provide valuable insights into brain function, cognitive states, and can aid in diagnosing neurological disorders, studying sleep patterns, and exploring various aspects of brain activity. EEG technology is widely used in clinical settings, research laboratories, and emerging applications such as brain-computer interfaces.
Next, let's dive into the real-world applications of EEG.
Clinical Diagnostics: EEG's Role in Unraveling Brain Patterns
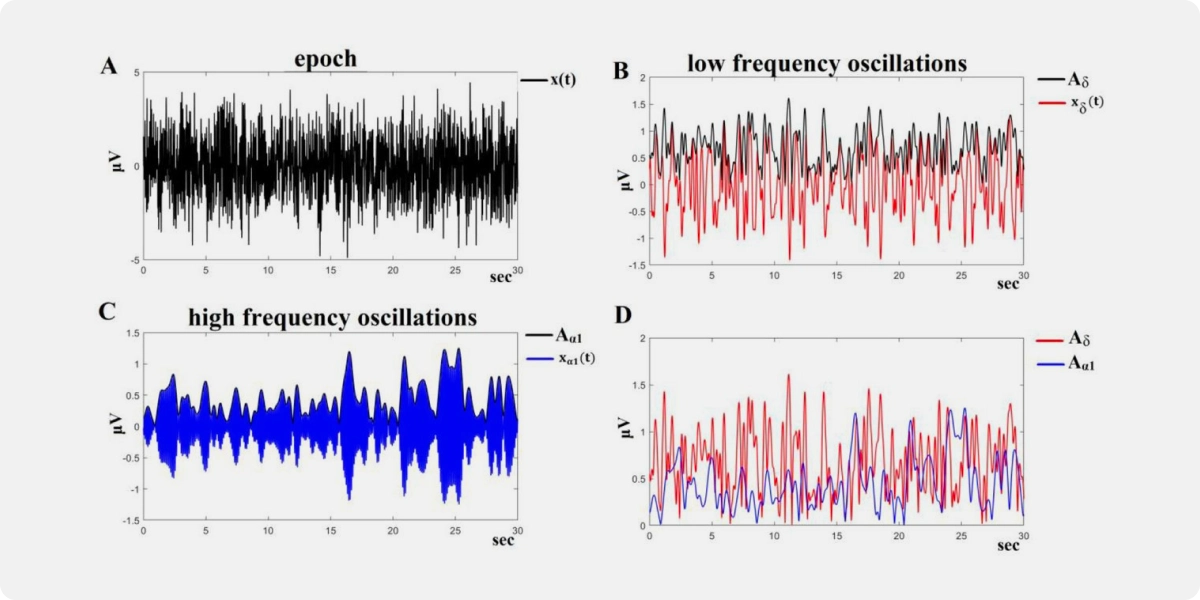
Epilepsy Monitoring: Precision in Seizure Identification
EEG technology, equipped with strategically placed electrodes on the scalp, proves indispensable in capturing and identifying abnormal electrical patterns indicative of seizures. Its role extends beyond observation, becoming a crucial tool in determining optimal strategies for treating epilepsy.
Sleep Disorders: Polysomnography's Contribution to Diagnosis
Polysomnography, a comprehensive sleep study incorporating EEG, serves as a meticulous observer of brain activity during different sleep stages. Beyond observation, EEG takes a leading role in conducting a detailed analysis essential for diagnosing a spectrum of sleep disorders, from sleep apnea to insomnia.
Neurological Research: Navigating Cognitive Processes
Cognitive Neuroscience: ERPs and Temporal Precision
In cognitive neuroscience, EEG is an active participant, measuring Event-Related Potentials (ERPs) with exceptional temporal precision. The P300 waveform, reflecting attention and memory processing, empowers researchers to investigate cognitive phenomena with unparalleled detail.
Motor Control Studies for Neuroplasticity
Within motor control studies, EEG is instrumental in decoding the brain's role in planned or imagined movements. By capturing brain activity during motor imagery tasks, researchers gain insights into neuroplasticity, laying the groundwork for advancements in prosthetics and rehabilitation technologies.
Understanding Brainwave Frequencies to Optimise Performance via Cognitive Enhancement
Techniques like entrainment and binaural beats offer insights into the frequencies governing focus and learning. Unveiling the manipulation potential within these frequencies provides researchers with valuable insights that may shape interventions for cognitive improvement. This knowledge has the power to redefine methods in cognitive research, echoing the rhythm of the brainwave symphony.
Brain-Computer Interfaces (BCIs): Enabling Mind-Machine Interaction
Assistive Technology: Interpreting Motor Imagery Commands
EEG-based BCIs serve as a vital link between the mind and external devices. By detecting motor imagery or evoked potentials associated with specific commands, individuals with severe motor impairments gain the ability to control external devices. Signal processing algorithms play a crucial role in interpreting EEG data, translating mental intentions into actionable commands.
Neurofeedback and Cognitive Enhancement
Neurofeedback Therapy: Real-Time Modulation
In the therapeutic realm, EEG becomes a tool for real-time modulation of brain activity in neurofeedback therapy. EEG's real-time monitoring helps study how individuals consciously control their brain activity. Identifying specific frequency bands, like elevated theta power and nuanced alpha activity in ADHD, opens doors for targeted interventions. This could reshape how researchers approach conditions like anxiety, ADHD, and insomnia, creating tailored solutions for complex neuro challenges.
Cognitive Enhancement: Leveraging Brainwave Frequencies
Techniques like entrainment leverage EEG data to synchronize auditory or visual stimuli with specific brainwave frequencies. This unveils the manipulation potential within these frequencies, providing profound insights for researchers and practitioners. The aim is to enhance cognitive functions by entraining brainwave patterns associated with optimal performance.
Mental Health Diagnosis and Treatment: Mapping Brain Activity
Psychiatric Disorders: qEEG Analysis for Biomarker Identification
Quantitative EEG (qEEG) analysis introduces a new dimension to mapping brain activity in specific regions. This detailed mapping allows for the identification of aberrant patterns associated with psychiatric disorders. Increased theta or delta power serves as biomarkers, aiding in diagnoses and monitoring treatment efficacy.
Treatment Monitoring: Tracking Progress in Psychiatric Interventions
In psychiatric interventions, EEG frequency band analysis becomes a trusted companion for researchers. Changes in specific frequency bands, meticulously tracked over time, serve as compass points. These indicators offer valuable insights into treatment responses and the progression of psychiatric disorders, fostering a deeper understanding for effective treatment strategies.
Sleep Research: A look into Sleep Disorders
Monitoring delta and theta waves through EEG is crucial for advancing our understanding of sleep disorders. Researchers use EEG markers to explore sleep quality, diagnose disorders, and understand the connections between sleep and mental well-being. Specific EEG patterns correlate with conditions like borderline personality disorder, Rett syndrome, Asperger syndrome, respiratory failure, chronic fatigue, PTSD, and insomnia, opening rich avenues for exploration in sleep studies.
Integrating EEG with Advanced Technologies
The fusion of EEG with advanced technologies, especially artificial intelligence (AI), opens new frontiers for researchers. Applying machine learning algorithms to extensive EEG datasets has the potential to reveal intricate patterns and correlations, creating a symphony of synergy. This collaboration significantly amplifies the precision of diagnoses and treatment plans, propelling neuroscientific research into an era of profound discovery.
As researchers explore EEG applications, ethical considerations take centre stage. Privacy concerns, data security, and responsible handling of neurological information become critical. Researchers, much like skilled navigators, must traverse these ethical waters with discernment, ensuring the judicious and ethical use of EEG technologies in their studies.
In Conclusion
Electroencephalography (EEG) stands as an indispensable tool for researchers in neuroscience. Its applications span from delicate explorations into brain activity regulation to cognitive enhancement, sleep research, mental health diagnostics, and the integration with advanced technologies. As researchers continue to unravel the tapestry of the brain, EEG remains a resounding instrument, opening new avenues in our search for a deeper, more profound understanding of the mind.
Resources & further reading:
NCBI - WWW Error Blocked Diagnostic
NCBI - WWW Error Blocked Diagnostic
EEG Frequency Bands in Psychiatric Disorders: A Review of Resting State Studies
https://www.sciencedirect.com/science/article/pii/S0010482523001415
https://www.sciencedirect.com/science/article/pii/S0035378721006974
(PDF) Influence of Binaural Beats on EEG Signal
https://www.sciencedirect.com/science/article/pii/S1878929323001172
NCBI - WWW Error Blocked Diagnostic
Sleep Quality and Electroencephalogram Delta Power
Sleep EEG for Diagnosis and Research | Bitbrain
(PDF) Sleep Quality and Electroencephalogram Delta Power
NCBI - WWW Error Blocked Diagnostic
https://link.springer.com/article/10.1007/s10489-023-04702-5
EEG Frequency Bands in Psychiatric Disorders: A Review of Resting State Studies
Potential diagnostic biomarkers for schizophrenia
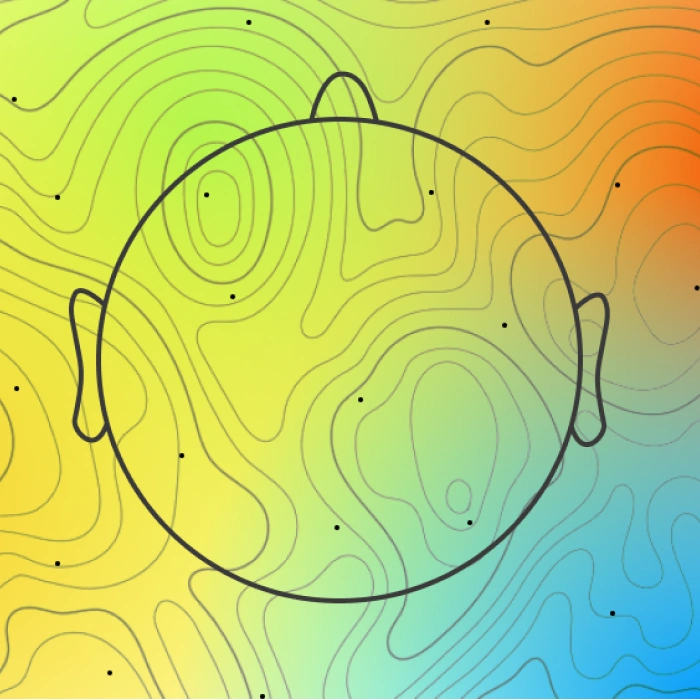
Journey through the brain's electromagnetic symphony, exploring how EEG frequency bands—from delta's deep rest to gamma's high-level cognition—reveal our mental states.
The human brain, with its intricate web of neurons, holds the key to understanding our thoughts, emotions, and behaviors. Within this complex neural network, various frequency bands of electromagnetic waves, as measured by EEG (Electroencephalography), give us insights into different states of consciousness and mental activities.
In this blog, we embark on a fascinating journey through these frequency bands, exploring their characteristics and potential applications.
Delta Waves: The Essence of Deep Rest
At less than 4 Hz, delta waves are the lullabies of our brain. They dominate during deep, dreamless sleep, providing a crucial window into our unconscious minds. Beyond sleep, delta waves can induce states of deep relaxation and trance, making them a powerful tool in practices like meditation and hypnotherapy
Theta Waves: Bridging Dreams and Reality
With frequencies ranging from 4 to 8 Hz, theta waves open the door to a realm between wakefulness and sleep. They flourish during meditation, prayer, and moments of spiritual awareness, fostering intuitive and creative thinking. These waves are the whispers of our subconscious mind, offering a path to enhanced focus and creativity.
Alpha Waves: The Symphony of Tranquility
Occurring at 8 to 12 Hz, alpha waves paint a picture of serenity. They signify a relaxed, yet conscious state, often experienced during meditation. By tapping into the power of alpha waves, we can attain inner-awareness, balance, and a deep sense of tranquility.
Beta Waves: The Spectrum of Awareness
Beta waves range from 12 to over 30 Hz, offering a spectrum of mental states. Low beta waves reflect relaxed focus, while mid beta waves denote alert mental activity. High beta waves signal heightened alertness and mental engagement. Understanding beta waves allows us to unlock our potential for focused attention, mental agility, and creative problem-solving.
Gamma Waves: The Orchestra of Integration
At frequencies surpassing 30 Hz, gamma waves represent the pinnacle of cognitive processing. They orchestrate high-level information integration and complex thought processes. While much is still to be discovered about gamma waves, their association with advanced mental tasks makes them a captivating area of study.

Unlocking Potential: Practical Applications
The rich tapestry of EEG frequency bands has practical applications that extend far beyond the realms of research. Cutting-edge technology now allows us to harness these waves for real-world use. Focus detection, calm detection, and concentration detection are just a few examples of how EEG data can be leveraged to enhance performance, well-being, and mental health.
How Nexstem helps?
Stream App's Bandpower Graph, which displays real-time EEG frequency band data, provides a powerful tool for monitoring brain activity in real-time. On the other hand, NexStem's WisdomAPI and WisdomSDK come equipped with advanced algorithms for Focus Detection, Emotion Detection, and Concentration, showcasing a comprehensive suite of capabilities for in-depth EEG data analysis.
This combination of real-time monitoring and advanced analytical tools sets a solid foundation for a wide range of applications in EEG research and development.
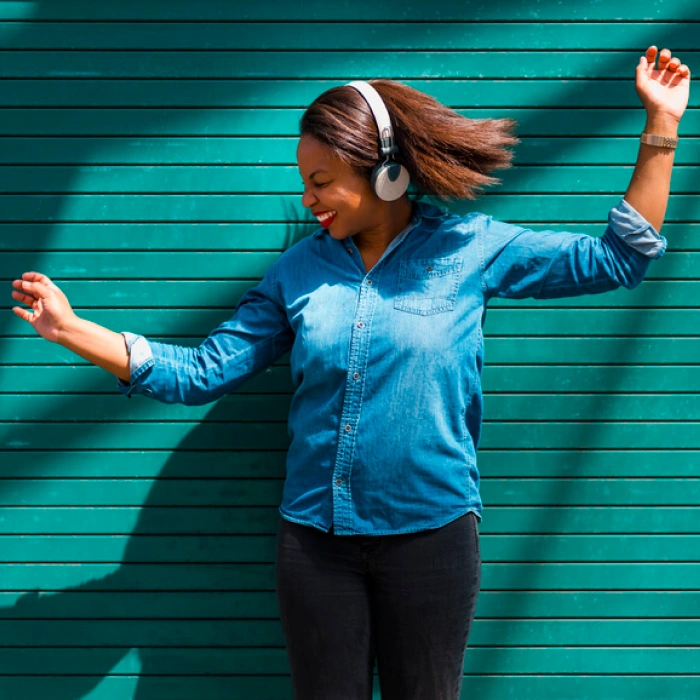
Learning to play a musical instrument not only enhances your musical skills but also reshapes the adult brain. Discover how musical training bridges nature and nurture, transforming both brain structure and function.
Music has long been known to have a profound impact on our emotions and well-being. But did you know that learning to play a musical instrument can also shape the adult brain? In a recent review article, researchers delve into the structural and functional differences between the brains of musicians and non-musicians, shedding light on the fascinating effects of musical training.
Nature vs. Nurture: Predispositions or Training?
One of the key questions in this inquiry is whether the observed differences between musicians and non-musicians are due to inherent predispositions or the result of training. Recent research explores brain reorganization and neuronal markers related to learning to play a musical instrument. Turns out, the "musical brain" is influenced by both natural human neurodiversity and training practice.
Structural and Functional Differences
There are structural and functional differences between the brains of musicians and non-musicians. Specifically, regions associated with motor control and auditory processing show notable disparities. These differences suggest that musical training can lead to specific adaptations in these brain areas, potentially enhancing motor skills and auditory perception.
Impact on the Motor Network and Auditory System
Longitudinal studies have demonstrated that music training can induce functional changes in the motor network and its connectivity with the auditory system. This finding suggests that learning to play an instrument not only refines motor control but also strengthens the integration between auditory and motor processes. Such cross-modal plasticity may contribute to musicians' exceptional ability to synchronize their movements with sound.

Predictors of Musical Learning Success
Research has also found potential predictors of musical learning success. Specific brain activation patterns and functional connectivity are possible indicators of an individual's aptitude for musical training. These findings open up exciting possibilities for personalized approaches to music education, allowing educators to tailor instruction to each student's unique neural profile.
Some generic predictors, however, are:
Attitude and Motivation
Positive attitudes towards the music being learned and high motivational levels have emerged as significant predictors of musical learning success. Individuals displaying enthusiasm and a receptive mindset exhibit enhanced learning outcomes, underscoring the importance of psychological factors in the musical learning process.
Intelligence
General intelligence demonstrates a positive correlation with musical skill acquisition, suggesting that cognitive aptitude plays a pivotal role in mastering musical elements. This finding underscores the cognitive demands of musical learning and emphasizes the relevance of intelligence as a predictor of success in this domain.
Reward and Pleasure
The level of liking or enjoyment of a particular piece of music before training has been identified as a critical predictor influencing the ability to learn and achieve proficiency. The intrinsic reward and pleasure associated with musical engagement contribute to heightened receptivity and commitment to the learning process.
Music Predictability
Musical predictability emerges as a noteworthy factor influencing pupil dilation and promoting motor learning in non-musicians. The predictability of musical elements contributes to a more efficient cognitive processing of auditory information, enhancing the overall learning experience.
In conclusion, musical training has transformative effects on the adult brain. The differences observed between musicians and non-musicians are likely a result of a combination of innate predispositions and training practice, and understanding these neural adaptations can inform educational strategies and promote the benefits of music in cognitive development and overall well-being
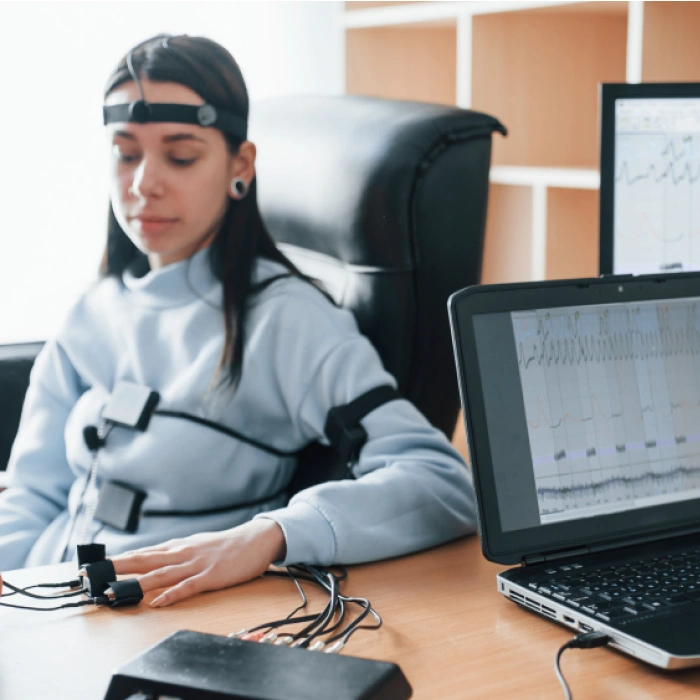
Did you know that it is possible to for a person to pass a lie detection test by exerting control over their physiological responses. Here we explore what would be an alternative to the common polygraph lie detection test using BCI technology.
Lie detection tests, often portrayed in movies as dramatic showdowns, are actually fascinating tools used in real-life scenarios. The most common method, the polygraph test, measures physiological responses like heart rate, blood pressure, and skin conductivity to assess truthfulness. While it's not foolproof and relies on the assumption that lying induces detectable physiological changes, it can be surprisingly accurate. The examiner sets the baseline by asking innocuous questions, then delves into the more critical queries. It's like a high-stakes game of poker, where involuntary reactions become the telltale signs. The results are akin to a puzzle for seasoned professionals, decoding the body's subtle cues to separate fact from fiction.
But there is a catch. It is possible for a person to potentially pass a lie detection test by exerting control over their physiological responses. This can be achieved through various techniques such as controlled breathing, mental distraction, or even the use of countermeasures like imagining stressful situations during baseline questions. Skilled individuals who are aware of these techniques may attempt to manipulate the results of the test. Additionally, some individuals may naturally exhibit limited physiological responses even when lying, making them more challenging to detect. So, despite its intriguing potential, lie detection tests aren't infallible and require skilled interpretation. They serve as one piece of the puzzle in investigations, reminding us that even in the quest for truth, human intuition and analysis remain paramount.
Electroencephalography (EEG)
It can be a more reliable alternative to polygraph tests. One may have semi-voluntary control over their physiological responses, but many internal mental responses are involuntary in nature. These responses can be reliably captured, and then recognized as patterns in EEG data. The P300 is one such pattern that can be used in lie-detection tests. All we need is a carefully designed environment, EEG recording setup, our prime suspect, and an invigilator - which could be another human or a simple computer program.
Picture P300 as your mental drum-roll, happening about 300 milliseconds after something catches your brain's eye. Now, here's the fun part: The brain throws this P300 party with a twist called the "oddball paradigm." It's like serving up a mix of familiar and surprise treats to your brain. When that surprise treat pops up, the P300 struts onto the scene, stealing the show with its snazzy moves! This P300 sensation isn't just for kicks though! It's your brain's secret agent, helping you focus on what really matters in a sea of distractions. It's like having a personal brain butler that whispers, "Hey, pay attention to this!"

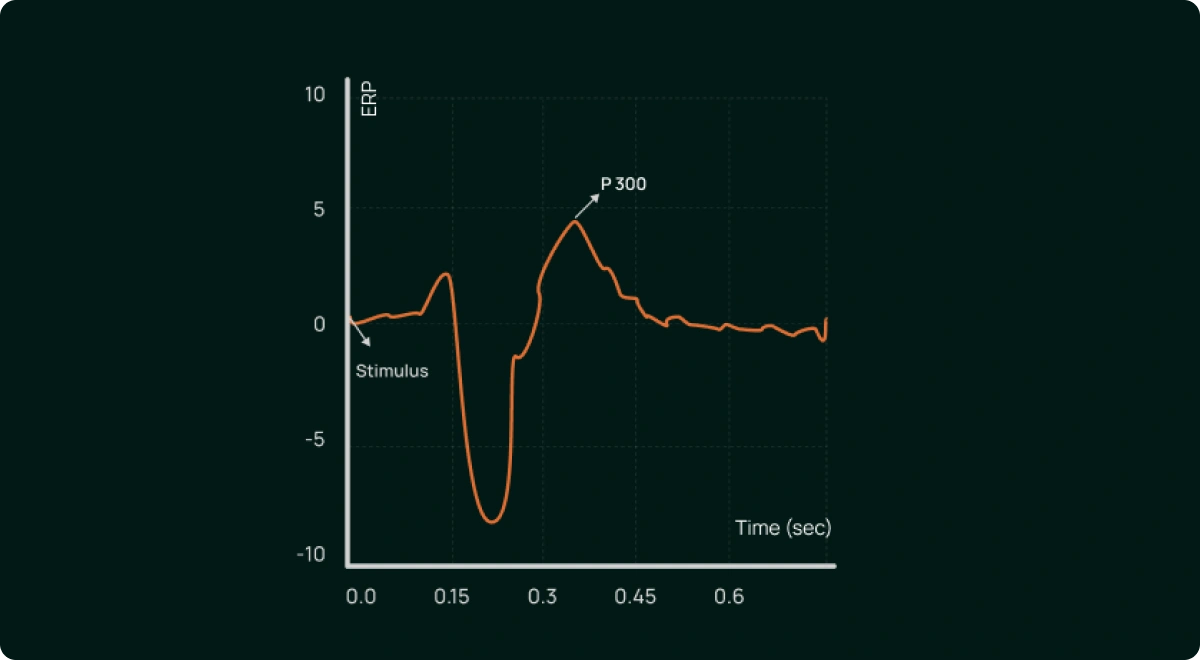
Role of P300 EEG Patterns
Let’s Imagine a scenario in a police investigation room to see how we can use P300 EEG patterns. Detective Anderson is questioning a suspect, John, about a recent burglary. John maintains his innocence, but Detective Anderson has reasons to suspect otherwise. This is where the P300, our cognitive truth-seeker, comes into play.
Detective Anderson has a set of statements related to the crime. Among them, there's one crucial statement he believes holds the truth: the location of a hidden stash of stolen goods. This statement is intermixed with other neutral statements to form a series.
John is instructed to respond truthfully to all statements. However, when he hears the statement about the hidden stash, he experiences a slight cognitive hiccup. This is because his brain, even though he's trying to hide it, recognizes the statement as relevant and unexpected. The P300, our lie-detecting superhero, picks up on this subtle brainwave pattern.
Meanwhile, electrodes placed on John's scalp are recording his brain activity. The EEG machine diligently captures the electrical signals generated by John's brain in response to each statement. When the statement about the hidden stash is presented, the P300 response emerges about 300 milliseconds later.
Detective Anderson, relying on the expertise of trained analysts and specialized software, examines the EEG data. They focus on the P300 response specifically, looking for distinct patterns that indicate heightened cognitive processing associated with the relevant statement.
In this case, the P300 signal corresponding to the statement about the hidden stash exhibits a stronger and more pronounced waveform compared to the neutral statements. This heightened P300 response is a telltale sign that John's brain recognizes the statement as important, suggesting he likely has knowledge of the hidden goods.
This crucial information becomes a powerful tool for Detective Anderson. While it doesn't serve as definitive proof of guilt, it provides a significant lead. It prompts further investigation, potentially leading to the recovery of the stolen items and strengthening the case against John.
Remember, this is a fictional scenario for illustrative purposes. In reality, things are not as simplistic. We would still need careful experimental design, scientific data analysis, and expert interpretation.
Current State of Research
A. Advancements in Signal Processing and Machine Learning
Researchers have made strides in refining signal processing techniques and applying machine learning algorithms to improve the accuracy and reliability of P300-based lie detection.
B. Integration with Multimodal Techniques
Combining EEG with other neuro-imaging methods (e.g., fMRI, eye-tracking) has shown promise in enhancing the accuracy of lie detection by providing complementary information.
C. Applied in Specific Contexts
P300-based lie detection has been explored in various domains, including criminal investigations, security screenings, and clinical assessments. It's important to note that it's not yet widely accepted for legal or forensic use in many jurisdictions.
D. BCIs and Assistive Technology
Beyond lie detection, the P300 has found applications in Brain-Computer Interfaces (BCIs), enabling individuals with motor disabilities to communicate or interact with their environment.
E. Potential Clinical Applications
P300-based research is extending into clinical areas, such as assessing cognitive functions in patients with brain injuries or neuro-degenerative disorders.
Challenges:
1. Individual Variability
Brainwave patterns can vary widely among individuals. This variability poses a challenge in developing a universal lie detection model that applies to all.
2. Ethical and Legal Considerations
The admissibility of P300-based lie detection in legal settings remains a subject of debate. False positives and negatives can have significant consequences, so ethical and legal frameworks must be carefully considered.
3. Real-World Context and Stress
Laboratory experiments may not fully capture the complexity and stress of real-world situations, where emotions, distractions, and high-stakes scenarios can influence results.
4. Interpretation of Results
While the P300 provides valuable information, interpreting its presence or absence requires expert knowledge and careful consideration of experimental design.
5. Cost and Accessibility
EEG equipment and expertise in analysis can be expensive and require specialized training, limiting the accessibility of P300-based lie detection methods.
6. Continual Technological Advancements
The field of EEG and lie detection is rapidly evolving. Keeping up with the latest technology and methodologies is crucial for accurate and reliable results.
In summary, while P300-based lie detection holds promise, it's not without its challenges. Ongoing research and advancements in technology, coupled with careful consideration of ethical and legal implications, are essential in moving this field forward.
Further reading:
- For deep dive into the P300 pattern -
The P300 Wave of the Human Event-Related Potential - P300 based lie detection-
Evaluation of P300 based Lie Detection Algorithm
P300 Based Deception Detection Using Convolutional Neural Networks
An experiment of lie detection based EEG-P300 classified by SVM algorithm - Other ways of lie detection using EEG-
Truth Identification from EEG Signal by using Convolution neural network: Lie Detection
Truth Identification from EEG Signal Using Frequency and Time Features with SVM Classifier

In the exciting world of neuroscience, the collaboration of BCI technology with AI steers in a promising phase of expansion and development. At Nexstem, we are at the forefront of this revolution.
In the exciting world of neuroscience, the collaboration of Brain-Computer Interface (BCI) technology with Artificial Intelligence (AI) steers in a promising phase of expansion and development. At Nexstem, we are at the forefront of this revolution, leveraging cutting-edge hardware and software to unlock the full potential of BCI systems. Let's take a journey as we delve into how AI is changing the landscape of BCI technology and the remarkable impact it holds for the destiny of neuroscience.
Introduction to BCI and AI
A Brain-Computer Interface (BCI) is a technology that facilitates direct communication between the brain and external devices, allowing for control or interaction without needing physical movement. In contrast, AI boosts devices to gain knowledge from data, adjust to new information, and carry out tasks smartly. When combined, BCI and AI chart a course for ground-breaking applications that revolutionize the interaction between humans and machines.
Integrating AI into BCI System
AI-based methods including machine learning, deep learning, and neural networks have been thoroughly blended into BCI systems, ramping up their utility, effectiveness, and user-friendliness. The power of AI algorithms allows BCI systems to decode intricate brain signals, cater to individual user needs, and fine-tune system engagements on the fly.
One such example is the combination of machine learning algorithms, particularly deep learning methods, with EEG-based BCIs for motor imagery tasks.
Motor imagery involves imagining the movement of body parts without physically executing them. EEG signals recorded during motor imagery tasks contain patterns that correspond to different imagined movements, such as moving the left or right hand. By training deep learning models, such as convolutional neural networks (CNNs) or recurrent neural networks (RNNs), with large datasets of EEG recordings from motor imagery experiments, researchers can develop highly accurate classification algorithms capable of decoding these intricate brain signals.
For instance, studies have shown that CNNs trained on EEG data can achieve remarkable accuracy in classifying motor imagery tasks, enabling precise control of BCI-driven devices like prosthetic limbs or computer cursors. Furthermore, incorporating techniques like transfer learning, where pre-trained CNN models are fine-tuned on smaller, task-specific datasets, can facilitate the adaptation of BCI systems to individual user preferences and neurophysiological characteristics.
Moreover, advancements in reinforcement learning algorithms offer opportunities to dynamically adjust BCI parameters based on real-time feedback from users. By continuously learning and adapting to user behavior, reinforcement learning-based BCI systems can optimize system engagements on the fly, enhancing user experience and performance over time.
Signal Processing and Analysis
Artificial Intelligence is instrumental in the world of signal processing and analysis when it comes to Brain-Computer Interface systems. It uses cutting-edge algorithms for specific feature extraction, sorting brain signals, and removing unnecessary noise, all of which make the data collected more accurate and trustworthy. These data yield critical understanding about brain functioning, opening doors for myriad applications.
Specific algorithms are commonly employed for various tasks in signal processing, particularly in feature extraction.
Feature Extraction Algorithms
Advanced signal processing algorithms such as Common Spatial Patterns (CSP), Time-Frequency Analysis (TFA), and Independent Component Analysis (ICA) are extensively utilized for precise feature extraction in BCI systems. These algorithms are specifically designed to identify and extract relevant patterns in brain signals associated with specific mental tasks or intentions.
Noise Reduction Techniques
Despite their effectiveness, BCI systems often encounter various types of noise, including electrical interference, muscle activity artifacts, and environmental factors. To ensure the integrity of neural signals, sophisticated noise reduction techniques are employed.
Types of Noise and Mitigation Techniques
Electrical Interference: Adaptive filtering techniques are employed to suppress electrical interference from surrounding equipment.
Muscle Activity Artifacts: Artifact removal algorithms, such as Independent Component Analysis (ICA), are utilized to eliminate muscle activity artifacts from the recorded signals.
Environmental Factors: Spatial filtering methods like Common Spatial Patterns (CSP) are implemented to mitigate the impact of environmental noise.
Ensuring Data Quality
These noise reduction techniques are crucial for maintaining the quality and reliability of the collected data, ensuring that it is suitable for subsequent analysis and interpretation. By effectively suppressing unwanted noise, BCI systems can provide accurate and trustworthy data for various applications.
Adaptive and Intelligent Interfaces
The role of AI is crucial in creating intelligent and customizable interfaces for BCI systems. It ensures a personalized, responsive, and predictive modeling based on user habits. These interfaces significantly improve user involvement, productivity, and satisfaction in numerous applications.
Let's delve into a case study that exemplifies the fusion of AI and BCI technology
Primary Technology
The Crown, a specialized EEG headset, focuses on BCIs employing EEG technology for real-time cognitive state monitoring and interaction.
Use Case(s)
The Crown utilizes machine learning algorithms to interpret EEG data, providing actionable metrics on cognitive states such as focus and emotional well-being. Designed for both consumers and developers, it interfaces with various platforms, serving diverse use cases from productivity enhancement to research.
Example Experiences
1. Music Shift
Music Shift utilizes The Crown's EEG capabilities to measure the brain's response to music, identifying songs that enhance concentration. The app connects with Spotify Premium accounts to curate playlists that maintain focus and promote a flow state.
2. Mind-controlled Dino game (Created by Charlie Gerard)
This project leverages The Crown to train specific thoughts, like tapping the right foot, to control actions in Chrome's Dino game. By interpreting EEG signals, users can interact with the game solely through their brain activity.
3. Brain-controlled Coffee Machine (Created by Wassim Chegham)
Using the Notion 2 headset, this project detects thoughts of moving the left index finger, triggering a coffee machine to brew and serve an Espresso via Bluetooth Low Energy (BLE). The integration of BCI technology allows users to control devices through their brain signals, enhancing convenience and accessibility.
In summary, The Crown exemplifies the integration of AI and BCI technology to create adaptive and intelligent interfaces. By leveraging machine learning algorithms and EEG technology, it enables a range of innovative experiences, from enhancing concentration with personalized music playlists to controlling devices through brain signals, ultimately improving user engagement and satisfaction.
Enhanced User Experience
BCI systems powered by AI play a vital role in augmenting user interaction by offering intuitive controls, minimizing mental burden, and encouraging more natural paradigms of interaction. Users can effortlessly undertake complex tasks and liaise with external devices, paving the way for a mutually beneficial partnership between humans and machines.
For instance, one example of intuitive controls is brain-controlled cursors, where users can move a cursor on a screen simply by imagining the movement of their limbs. This approach eliminates the need for traditional input devices like mice or touchpads, reducing physical effort and cognitive load for users.
Another intuitive control mechanism is the use of predictive typing interfaces, where AI algorithms analyze users' brain signals to anticipate their intended words or phrases. By predicting users' inputs, these interfaces can speed up the typing process and alleviate the cognitive burden associated with manual typing, particularly for individuals with motor impairments.
Furthermore, gesture recognition systems, integrated with AI algorithms, enable users to control devices through natural hand movements or gestures detected by wearable sensors. By translating hand gestures into commands, these systems offer a more intuitive and expressive means of interaction, resembling natural human communication.
Improving Performance and Accuracy
Artificial Intelligence (AI) is essential in enhancing the efficiency and precision of Brain-Computer Interface (BCI) systems by leading the progress in decoding algorithms, error rectification methods, and adaptive learning models. By ceaselessly learning from user responses and refining the dissection of data, AI endows BCIs to attain unparalleled degrees of detail and dependability.
Applications in Healthcare and Rehabilitation
Healthcare and rehabilitation procedures are being revolutionized by AI-enhanced BCI systems. This shift encompasses assistive technology, neurorehabilitation, and the diagnosis of brain-related conditions. These systems present innovative methods for enhancing health results and standard of living, laying a foundation for individualized and evidence-based strategies
Challenges and Future Directions
Despite AI's enormous promise in BCI creation, there are still periods of difficulty yet to be navigated, encompassing issues like the acquisition and utilization of brain data, comprehension capabilities, and ethical questions. One of the main challenges lies in the availability and quality of brain data required for training AI algorithms in BCI systems. Access to large, diverse, and well-curated datasets is essential for developing accurate and robust models capable of decoding complex brain signals effectively.
Furthermore, ethical considerations surrounding the collection, storage, and usage of brain data present significant challenges in the field of AI-powered BCIs. Safeguarding user privacy, ensuring informed consent, and addressing concerns related to data security and potential misuse are paramount. The ethical implications of BCI technology extend beyond individual privacy to broader societal concerns, including the potential for discrimination, surveillance, and unintended consequences.
Tackling these hurdles and outlining the path ahead for exploration, as well as innovation, is crucial for unlocking the comprehensive potential of AI-powered BCI systems and progressing within the neuroscience domain. Addressing the challenges of brain data acquisition and ethical considerations not only facilitates the development of more reliable and ethically responsible BCI technologies but also fosters trust and acceptance among users and stakeholders. By prioritizing ethical principles and responsible practices, the BCI community can pave the way for the ethical and equitable deployment of AI-driven neurotechnologies in diverse applications, from healthcare to assistive technology and beyond.
Conclusion
In the world of neuroscience and technology, combining Brain-Computer Interface (BCI) with AI represents a remarkable convergence of human ingenuity and technological innovation. It's like bringing together our brains and technology to do amazing things. But as we explore this new frontier, it's important to remember to do it right.
We need to make sure we are using AI and BCI in ways that respect people's privacy and rights. By working together and being open about what we're doing, we can ensure that the benefits of BCI technology are accessible to all while safeguarding the privacy and dignity of individuals.
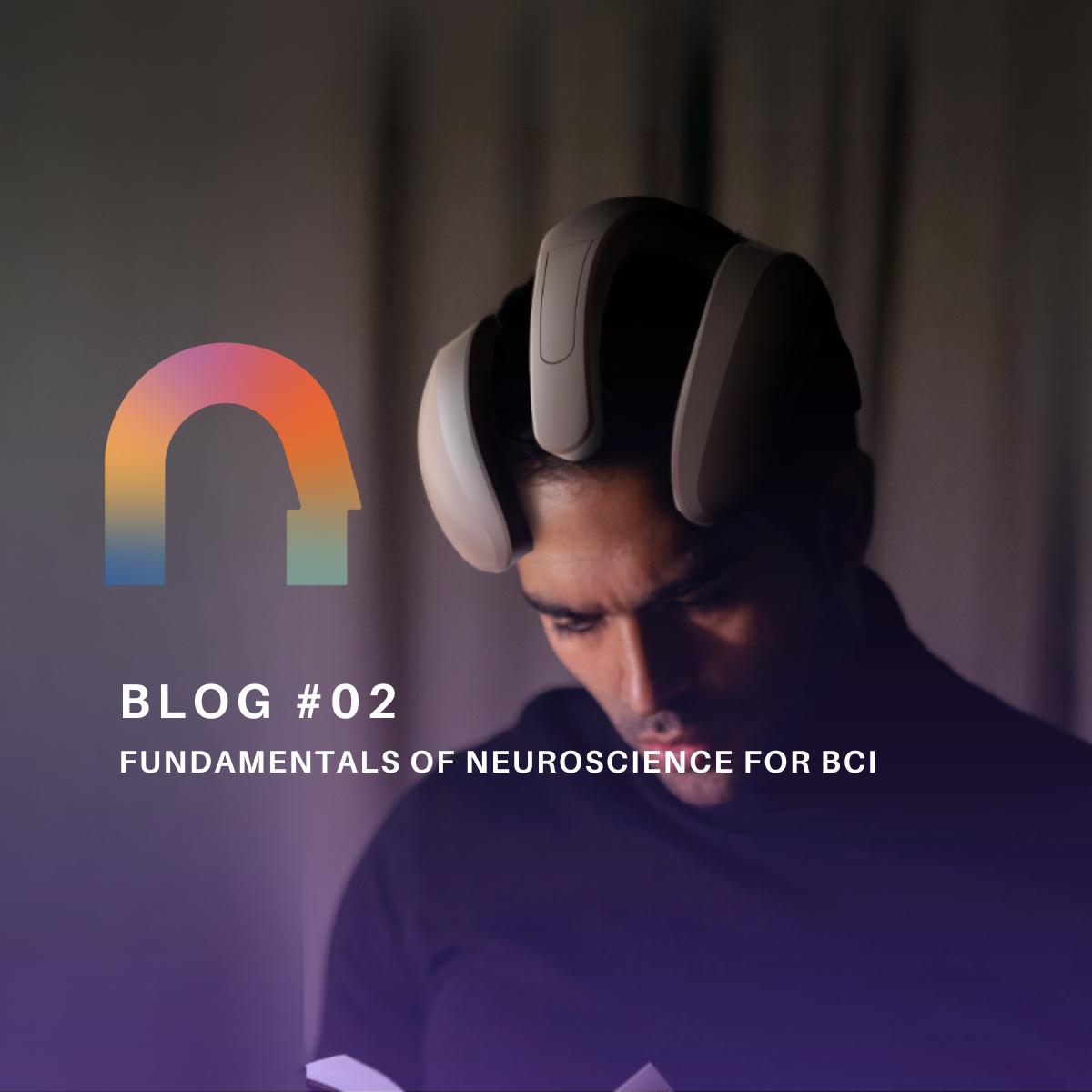
Lorem ipsum dolor sit amet, consectetur adipiscing elit. Suspendisse varius enim in eros elementum tristique. Duis cursus, mi quis viverra ornare, eros dolor interdum nulla, ut commodo diam libero vitae erat. Aenean faucibus nibh et justo cursus id rutrum lorem imperdiet. Nunc ut sem vitae risus tristique posuere.
.png)
Lorem ipsum dolor sit amet, consectetur adipiscing elit. Suspendisse varius enim in eros elementum tristique. Duis cursus, mi quis viverra ornare, eros dolor interdum nulla, ut commodo diam libero vitae erat. Aenean faucibus nibh et justo cursus id rutrum lorem imperdiet. Nunc ut sem vitae risus tristique posuere.
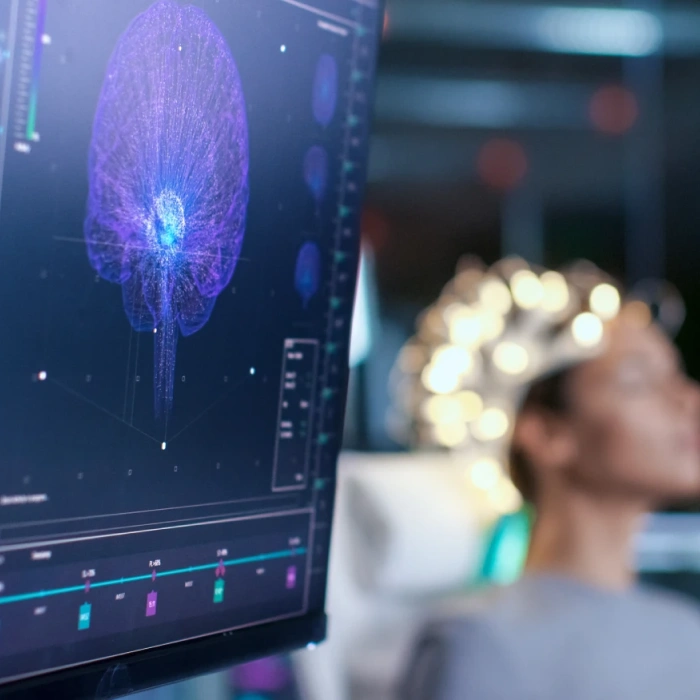
Lorem ipsum dolor sit amet, consectetur adipiscing elit. Suspendisse varius enim in eros elementum tristique. Duis cursus, mi quis viverra ornare, eros dolor interdum nulla, ut commodo diam libero vitae erat. Aenean faucibus nibh et justo cursus id rutrum lorem imperdiet. Nunc ut sem vitae risus tristique posuere.
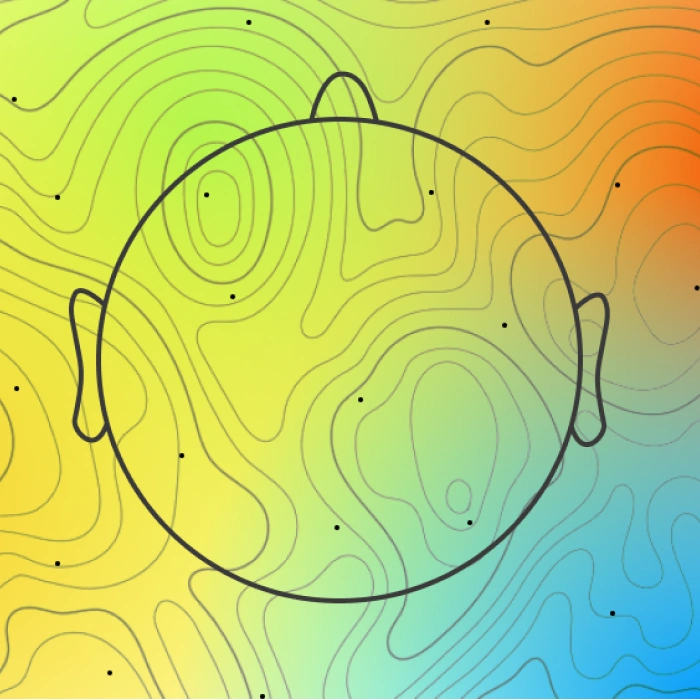
Lorem ipsum dolor sit amet, consectetur adipiscing elit. Suspendisse varius enim in eros elementum tristique. Duis cursus, mi quis viverra ornare, eros dolor interdum nulla, ut commodo diam libero vitae erat. Aenean faucibus nibh et justo cursus id rutrum lorem imperdiet. Nunc ut sem vitae risus tristique posuere.
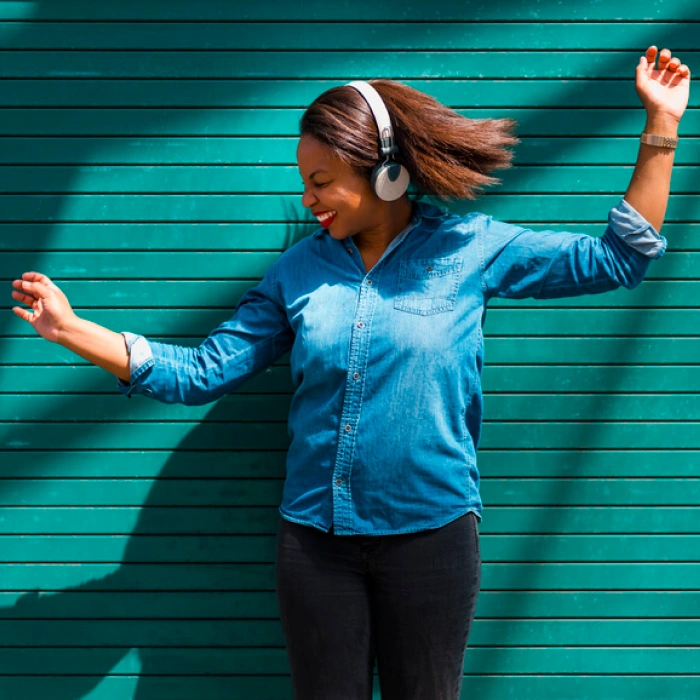
Lorem ipsum dolor sit amet, consectetur adipiscing elit. Suspendisse varius enim in eros elementum tristique. Duis cursus, mi quis viverra ornare, eros dolor interdum nulla, ut commodo diam libero vitae erat. Aenean faucibus nibh et justo cursus id rutrum lorem imperdiet. Nunc ut sem vitae risus tristique posuere.
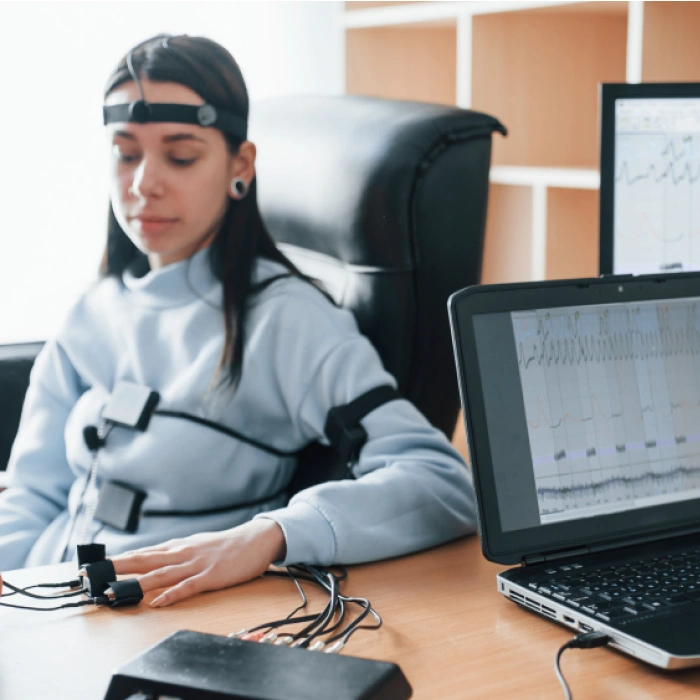
Lorem ipsum dolor sit amet, consectetur adipiscing elit. Suspendisse varius enim in eros elementum tristique. Duis cursus, mi quis viverra ornare, eros dolor interdum nulla, ut commodo diam libero vitae erat. Aenean faucibus nibh et justo cursus id rutrum lorem imperdiet. Nunc ut sem vitae risus tristique posuere.

Lorem ipsum dolor sit amet, consectetur adipiscing elit. Suspendisse varius enim in eros elementum tristique. Duis cursus, mi quis viverra ornare, eros dolor interdum nulla, ut commodo diam libero vitae erat. Aenean faucibus nibh et justo cursus id rutrum lorem imperdiet. Nunc ut sem vitae risus tristique posuere.
.webp)
.webp)
bci basics
BCI (Brain-Computer Interface) technology enables direct communication between the brain and external devices by interpreting brain signals and converting them into commands. This is generally achieved using a headset device such as Nexstem's Instinct headset which capture the brain signals and then processed it to make sense out of it predicting the users desired command.
This groundbreaking technology is used in various applications, including medical rehabilitation, gaming, and controlling prosthetic devices.
Yes, data from the Nexstem Instinct can be exported outside the device in various format including .xdf (Extensible Data Format) for further analysis and processing without any additional cost/fee. Our devices support Lab Streaming Layer (LSL) protocol, allowing seamless integration with various data analysis and visualization tools.
The Nexstem Instinct is suitable for a wide range of applications, including neurofeedback, brain-computer interface research, cognitive neuroscience, and in future clinical diagnostics. Its advanced features and high-quality data output make it ideal for both academic research and practical implementations.
stay in
the loop

Interact with Nexstem teams and join the vibrant community of neuroscience researchers, professors, innovators, developers and BCI enthusiasts.

Follow us on LinkedIn to get the latest news and developments from the world of BCI and Nexstem.

with us!
Follow us on LinkedIn to get the latest news and developments from the world of BCI and Nexstem.
do you have a
question for us?
Drop us your query and we will get back to you as soon as possible.

19, KHB Colony, Koramangala 8th Block, Bengaluru, Karnataka, India. 560095