The impact of musical training on the adult brain
Learning to play a musical instrument not only enhances your musical skills but also reshapes the adult brain. Discover how musical training bridges nature and nurture, transforming both brain structure and function.
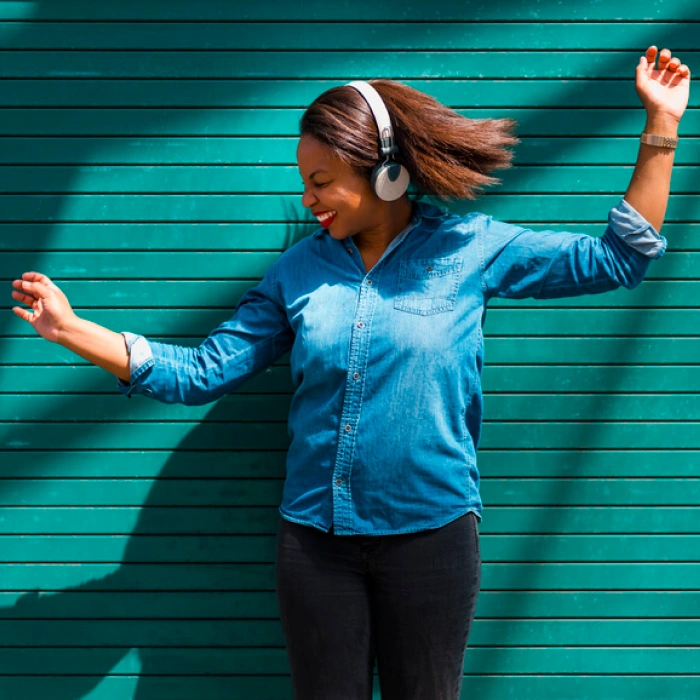
Music has long been known to have a profound impact on our emotions and well-being. But did you know that learning to play a musical instrument can also shape the adult brain? In a recent review article, researchers delve into the structural and functional differences between the brains of musicians and non-musicians, shedding light on the fascinating effects of musical training.
Nature vs. Nurture: Predispositions or Training?
One of the key questions in this inquiry is whether the observed differences between musicians and non-musicians are due to inherent predispositions or the result of training. Recent research explores brain reorganization and neuronal markers related to learning to play a musical instrument. Turns out, the "musical brain" is influenced by both natural human neurodiversity and training practice.
Structural and Functional Differences
There are structural and functional differences between the brains of musicians and non-musicians. Specifically, regions associated with motor control and auditory processing show notable disparities. These differences suggest that musical training can lead to specific adaptations in these brain areas, potentially enhancing motor skills and auditory perception.
Impact on the Motor Network and Auditory System
Longitudinal studies have demonstrated that music training can induce functional changes in the motor network and its connectivity with the auditory system. This finding suggests that learning to play an instrument not only refines motor control but also strengthens the integration between auditory and motor processes. Such cross-modal plasticity may contribute to musicians' exceptional ability to synchronize their movements with sound.

Predictors of Musical Learning Success
Research has also found potential predictors of musical learning success. Specific brain activation patterns and functional connectivity are possible indicators of an individual's aptitude for musical training. These findings open up exciting possibilities for personalized approaches to music education, allowing educators to tailor instruction to each student's unique neural profile.
Some generic predictors, however, are:
Attitude and Motivation
Positive attitudes towards the music being learned and high motivational levels have emerged as significant predictors of musical learning success. Individuals displaying enthusiasm and a receptive mindset exhibit enhanced learning outcomes, underscoring the importance of psychological factors in the musical learning process.
Intelligence
General intelligence demonstrates a positive correlation with musical skill acquisition, suggesting that cognitive aptitude plays a pivotal role in mastering musical elements. This finding underscores the cognitive demands of musical learning and emphasizes the relevance of intelligence as a predictor of success in this domain.
Reward and Pleasure
The level of liking or enjoyment of a particular piece of music before training has been identified as a critical predictor influencing the ability to learn and achieve proficiency. The intrinsic reward and pleasure associated with musical engagement contribute to heightened receptivity and commitment to the learning process.
Music Predictability
Musical predictability emerges as a noteworthy factor influencing pupil dilation and promoting motor learning in non-musicians. The predictability of musical elements contributes to a more efficient cognitive processing of auditory information, enhancing the overall learning experience.
In conclusion, musical training has transformative effects on the adult brain. The differences observed between musicians and non-musicians are likely a result of a combination of innate predispositions and training practice, and understanding these neural adaptations can inform educational strategies and promote the benefits of music in cognitive development and overall well-being
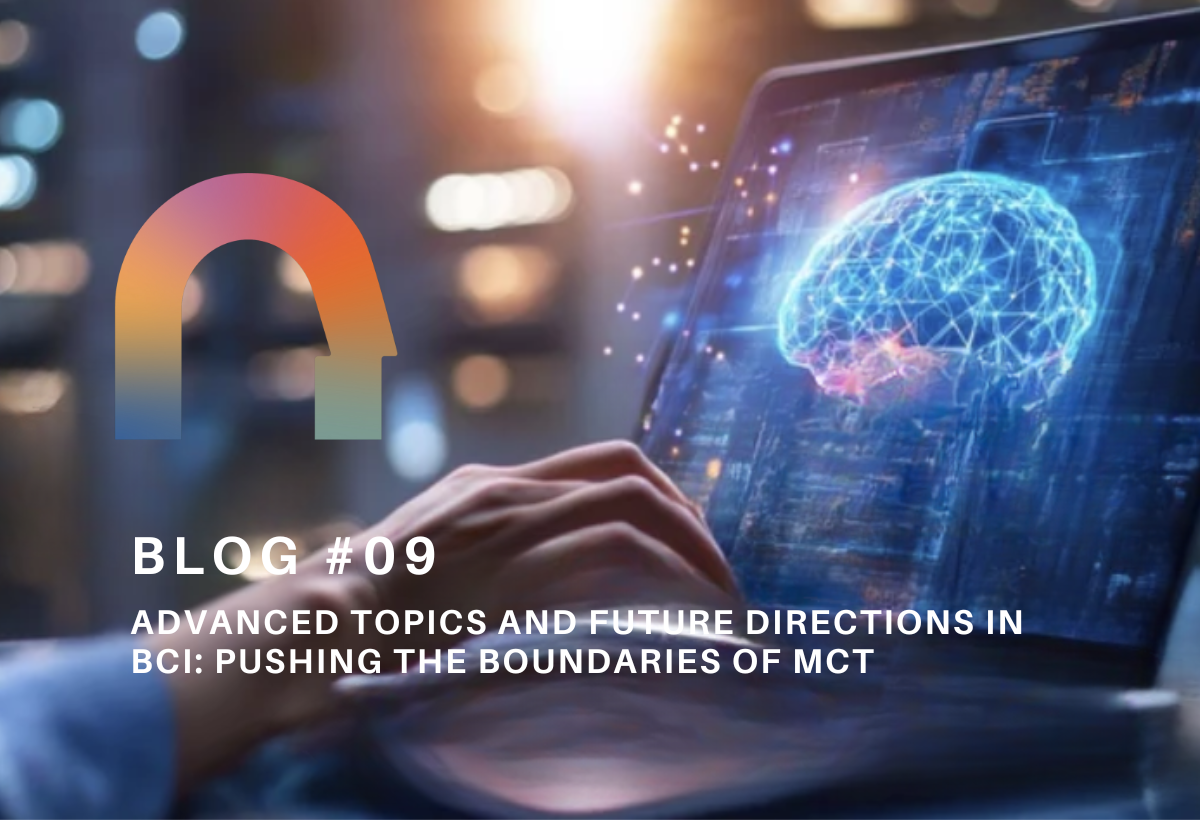
Welcome back to our BCI crash course! Over the past eight blogs, we have explored the fascinating intersection of neuroscience, engineering, and machine learning, from the fundamental concepts of BCIs to the practical implementation of real-world applications. In this final installment, we will shift our focus to the future of BCI, delving into advanced topics and research directions that are pushing the boundaries of mind-controlled technology. Get ready to explore the exciting possibilities of hybrid BCIs, adaptive algorithms, ethical considerations, and the transformative potential that lies ahead for this groundbreaking field.
Hybrid BCIs: Combining Paradigms for Enhanced Performance
As we've explored in previous posts, different BCI paradigms leverage distinct brain signals and have their strengths and limitations. Motor imagery BCIs excel at decoding movement intentions, P300 spellers enable communication through attention-based selections, and SSVEP BCIs offer high-speed control using visual stimuli.
What are Hybrid BCIs? Synergy of Brain Signals
Hybrid BCIs combine multiple BCI paradigms, integrating different brain signals to create more robust, versatile, and user-friendly systems. Imagine a BCI that leverages both motor imagery and SSVEP to control a robotic arm with greater precision and flexibility, or a system that combines P300 with error-related potentials (ErrPs) to improve the accuracy and speed of a speller.
Benefits of Hybrid BCIs: Unlocking New Possibilities
Hybrid BCIs offer several advantages over single-paradigm systems:
- Improved Accuracy and Reliability: Combining complementary brain signals can enhance the signal-to-noise ratio and reduce the impact of individual variations in brain activity, leading to more accurate and reliable BCI control.
- Increased Flexibility and Adaptability: Hybrid BCIs can adapt to different user needs, tasks, and environments by dynamically switching between paradigms or combining them in a way that optimizes performance.
- Richer and More Natural Interactions: Integrating multiple BCI paradigms opens up possibilities for creating more intuitive and natural BCI interactions, allowing users to control devices with a greater range of mental commands.
Examples of Hybrid BCIs: Innovations in Action
Research is exploring various hybrid BCI approaches:
- Motor Imagery + SSVEP: Combining motor imagery with SSVEP can enhance the control of robotic arms. Motor imagery provides continuous control signals for movement direction, while SSVEP enables discrete selections for grasping or releasing objects.
- P300 + ErrP: Integrating P300 with ErrPs, brain signals that occur when we make errors, can improve speller accuracy. The P300 is used to select letters, while ErrPs can be used to automatically correct errors, reducing the need for manual backspacing.
Adaptive BCIs: Learning and Evolving with the User
One of the biggest challenges in BCI development is the inherent variability in brain signals. A BCI system that works perfectly for one user might perform poorly for another, and even a single user's brain activity can change over time due to factors like learning, fatigue, or changes in attention. This is where adaptive BCIs come into play, offering a dynamic and personalized approach to brain-computer interaction.
The Need for Adaptation: Embracing the Brain's Dynamic Nature
BCI systems need to adapt to several factors:
- Changes in User Brain Activity: Brain signals are not static. They evolve as users learn to control the BCI, become fatigued, or shift their attention. An adaptive BCI can track these changes and adjust its processing accordingly.
- Variations in Signal Quality and Noise: EEG recordings can be affected by various sources of noise, from muscle artifacts to environmental interference. An adaptive BCI can adjust its filtering and artifact rejection parameters to maintain optimal signal quality.
- Different User Preferences and Skill Levels: BCI users have different preferences for control strategies, feedback modalities, and interaction speeds. An adaptive BCI can personalize its settings to match each user's individual needs and skill level.
Methods for Adaptation: Tailoring BCIs to the Individual
Various techniques can be employed to create adaptive BCIs:
- Machine Learning Adaptation: Machine learning algorithms, such as those used for classification, can be trained to continuously learn and update the BCI model based on the user's brain data. This allows the BCI to adapt to changes in brain patterns over time and improve its accuracy and responsiveness.
- User Feedback Adaptation: BCIs can incorporate user feedback, either explicitly (through direct input) or implicitly (by monitoring performance and user behavior), to adjust parameters and optimize the interaction. For example, if a user consistently struggles to control a motor imagery BCI, the system could adjust the classification thresholds or provide more frequent feedback to assist them.
Benefits of Adaptive BCIs: A Personalized and Evolving Experience
Adaptive BCIs offer significant advantages:
- Enhanced Usability and User Experience: By adapting to individual needs and preferences, adaptive BCIs can become more intuitive and easier to use, reducing user frustration and improving the overall experience.
- Improved Long-Term Performance and Reliability: Adaptive BCIs can maintain high levels of performance and reliability over time by adjusting to changes in brain activity and signal quality.
- Personalized BCIs: Adaptive algorithms can tailor the BCI to each user's unique brain patterns, preferences, and abilities, creating a truly personalized experience.
Ethical Considerations: Navigating the Responsible Development of BCI
As BCI technology advances, it's crucial to consider the ethical implications of its development and use. BCIs have the potential to profoundly impact individuals and society, raising questions about privacy, autonomy, fairness, and responsibility.
Introduction: Ethics at the Forefront of BCI Innovation
Ethical considerations should be woven into the fabric of BCI research and development, guiding our decisions and ensuring that this powerful technology is used for good.
Key Ethical Concerns: Navigating a Complex Landscape
- Privacy and Data Security: BCIs collect sensitive brain data, raising concerns about privacy violations and potential misuse. Robust data security measures and clear ethical guidelines are crucial for protecting user privacy and ensuring responsible data handling.
- Agency and Autonomy: BCIs have the potential to influence user thoughts, emotions, and actions. It's essential to ensure that BCI use respects user autonomy and agency, avoiding coercion, manipulation, or unintended consequences.
- Bias and Fairness: BCI algorithms can inherit biases from the data they are trained on, potentially leading to unfair or discriminatory outcomes. Addressing these biases and developing fair and equitable BCI systems is essential for responsible innovation.
- Safety and Responsibility: As BCIs become more sophisticated and integrated into critical applications like healthcare and transportation, ensuring their safety and reliability is paramount. Clear lines of responsibility and accountability need to be established to mitigate potential risks and ensure ethical use.
Guidelines and Principles: A Framework for Responsible BCI
Efforts are underway to establish ethical guidelines and principles for BCI research and development. These guidelines aim to promote responsible innovation, protect user rights, and ensure that BCI technology benefits society as a whole.
Current Challenges and Future Prospects: The Road Ahead for BCI
While BCI technology has made remarkable progress, several challenges remain to be addressed before it can fully realize its transformative potential. However, the future of BCI is bright, with exciting possibilities on the horizon for enhancing human capabilities, restoring lost function, and improving lives.
Technical Challenges: Overcoming Roadblocks to Progress
- Signal Quality and Noise: Non-invasive BCIs, particularly those based on EEG, often suffer from low signal-to-noise ratios. Improving signal quality through advanced electrode designs, noise reduction algorithms, and a better understanding of brain signals is crucial for enhancing BCI accuracy and reliability.
- Robustness and Generalizability: Current BCI systems often work well in controlled laboratory settings but struggle to perform consistently across different users, environments, and tasks. Developing more robust and generalizable BCIs is essential for wider adoption and real-world applications.
- Long-Term Stability: Maintaining the long-term stability and performance of BCI systems, especially for implanted devices, is a significant challenge. Addressing issues like biocompatibility, signal degradation, and device longevity is crucial for ensuring the viability of invasive BCIs.
Future Directions: Expanding the BCI Horizon
- Non-invasive Advancements: Research is focusing on developing more sophisticated and user-friendly non-invasive BCI systems. Advancements in EEG technology, including dry electrodes, high-density arrays, and mobile brain imaging, hold promise for creating more portable, comfortable, and accurate non-invasive BCIs.
- Clinical Applications: BCIs are showing increasing promise for clinical applications, such as restoring lost motor function in individuals with paralysis, assisting in stroke rehabilitation, and treating neurological disorders like epilepsy and Parkinson's disease. Ongoing research and clinical trials are paving the way for wider adoption of BCIs in healthcare.
- Cognitive Enhancement: BCIs have the potential to enhance cognitive abilities, such as memory, attention, and learning. Research is exploring ways to use BCIs for cognitive training and to develop brain-computer interfaces that can augment human cognitive function.
- Brain-to-Brain Communication: One of the most futuristic and intriguing directions in BCI research is the possibility of direct brain-to-brain communication. Studies have already demonstrated the feasibility of transmitting simple signals between brains, opening up possibilities for collaborative problem-solving, enhanced empathy, and new forms of communication.
Resources for Further Learning and Development
- Brain-Computer Interface Wiki
- Research Journals and Conferences:some text
- Journal of Neural Engineering: https://iopscience.iop.org/journal/1741-2560 - A leading journal for BCI research and related fields.
- Brain-Computer Interfaces: https://www.tandfonline.com/toc/tbci20/current - A dedicated journal focusing on advances in BCI technology and applications.
Embracing the Transformative Power of BCI
From hybrid systems to adaptive algorithms, ethical considerations, and the exciting possibilities of the future, we've explored the cutting edge of BCI technology. This field is rapidly evolving, driven by advancements in neuroscience, engineering, and machine learning.
BCIs hold immense potential to revolutionize how we interact with technology, enhance human capabilities, restore lost function, and improve lives. As we continue to push the boundaries of mind-controlled technology, the future promises a world where our thoughts can seamlessly translate into actions, unlocking new possibilities for communication, control, and human potential.
As we wrap up this course with this final blog article, we hope that you gained an overview as well as practical expertise in the field of BCIs. Please feel free to reach out to us with feedback and areas of improvement. Thank you for reading along so far, and best wishes for further endeavors in your BCI journey!
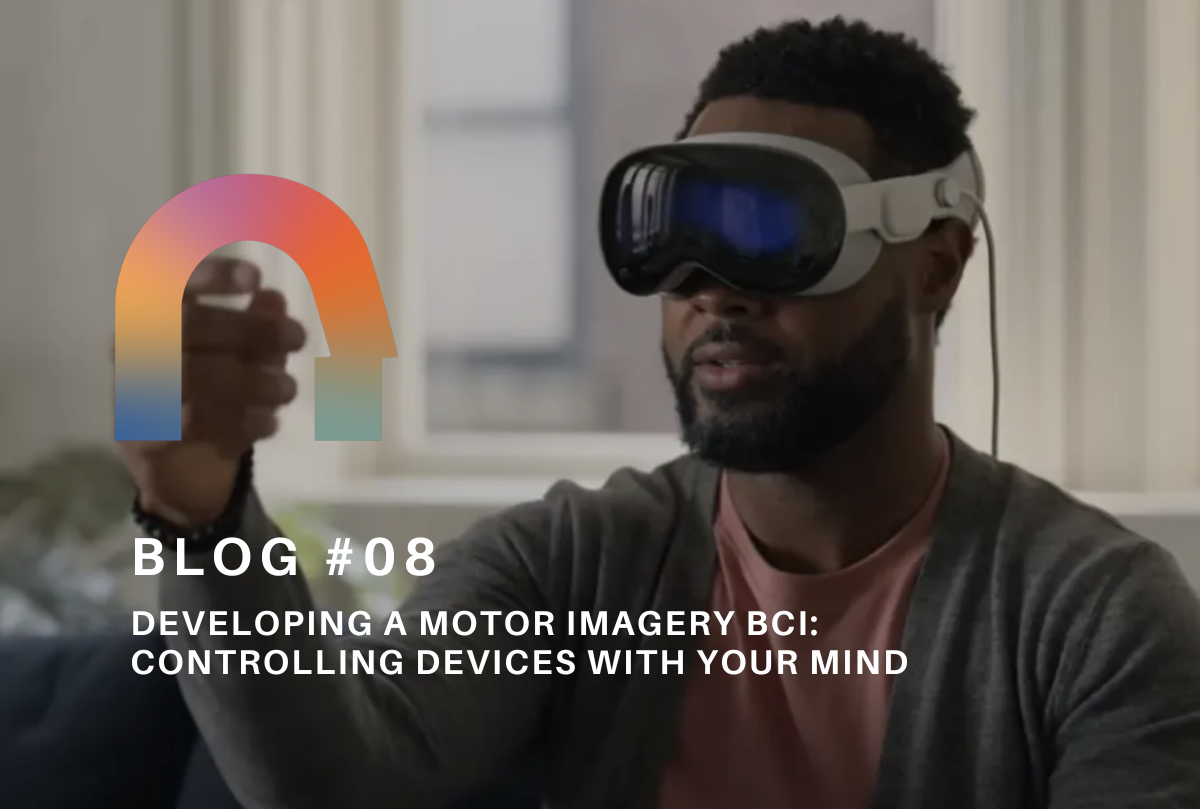
Welcome back to our BCI crash course! We've journeyed from the fundamental concepts of BCIs to the intricacies of brain signals, mastered the art of signal processing, and learned how to train intelligent algorithms to decode those signals. Now, we're ready to tackle a fascinating and powerful BCI paradigm: motor imagery. Motor imagery BCIs allow users to control devices simply by imagining movements. This technology holds immense potential for applications like controlling neuroprosthetics for individuals with paralysis, assisting in stroke rehabilitation, and even creating immersive gaming experiences. In this post, we'll guide you through the step-by-step process of building a basic motor imagery BCI using Python, MNE-Python, and scikit-learn. Get ready to harness the power of your thoughts to interact with technology!
Understanding Motor Imagery: The Brain's Internal Rehearsal
Before we dive into building our BCI, let's first understand the fascinating phenomenon of motor imagery.
What is Motor Imagery? Moving Without Moving
Motor imagery is the mental rehearsal of a movement without actually performing the physical action. It's like playing a video of the movement in your mind's eye, engaging the same neural processes involved in actual execution but without sending the final commands to your muscles.
Neural Basis of Motor Imagery: The Brain's Shared Representations
Remarkably, motor imagery activates similar brain regions and neural networks as actual movement. The motor cortex, the area of the brain responsible for planning and executing movements, is particularly active during motor imagery. This shared neural representation suggests that imagining a movement is a powerful way to engage the brain's motor system, even without physical action.
EEG Correlates of Motor Imagery: Decoding Imagined Movements
Motor imagery produces characteristic changes in EEG signals, particularly over the motor cortex. Two key features are:
- Event-Related Desynchronization (ERD): A decrease in power in specific frequency bands (mu, 8-12 Hz, and beta, 13-30 Hz) over the motor cortex during motor imagery. This decrease reflects the activation of neural populations involved in planning and executing the imagined movement.
- Event-Related Synchronization (ERS): An increase in power in those frequency bands after the termination of motor imagery, as the brain returns to its resting state.
These EEG features provide the foundation for decoding motor imagery and building BCIs that can translate imagined movements into control signals.
Building a Motor Imagery BCI: A Step-by-Step Guide
Now that we understand the neural basis of motor imagery, let's roll up our sleeves and build a BCI that can decode these imagined movements. We'll follow a step-by-step process, using Python, MNE-Python, and scikit-learn to guide us.
1. Loading the Dataset
Choosing the Dataset: BCI Competition IV Dataset 2a
For this project, we'll use the BCI Competition IV dataset 2a, a publicly available EEG dataset specifically designed for motor imagery BCI research. This dataset offers several advantages:
- Standardized Paradigm: The dataset follows a well-defined experimental protocol, making it easy to understand and replicate. Participants were instructed to imagine moving their left or right hand, providing clear labels for our classification task.
- Multiple Subjects: It includes recordings from nine subjects, providing a decent sample size to train and evaluate our BCI model.
- Widely Used: This dataset has been extensively used in BCI research, allowing us to compare our results with established benchmarks and explore various analysis approaches.
You can download the dataset from the BCI Competition IV website (http://www.bbci.de/competition/iv/).
Loading the Data: MNE-Python to the Rescue
Once you have the dataset downloaded, you can load it using MNE-Python's convenient functions. Here's a code snippet to get you started:
import mne
# Set the path to the dataset directory
data_path = '<path_to_dataset_directory>'
# Load the raw EEG data for subject 1
raw = mne.io.read_raw_gdf(data_path + '/A01T.gdf', preload=True)
Replace <path_to_dataset_directory> with the actual path to the directory where you've stored the dataset files. This code loads the data for subject "A01" from the training session ("T").
2. Data Preprocessing: Preparing the Signals for Decoding
Raw EEG data is often noisy and contains artifacts that can interfere with our analysis. Preprocessing is crucial for cleaning up the data and isolating the relevant brain signals associated with motor imagery.
Channel Selection: Focusing on the Motor Cortex
Since motor imagery primarily activates the motor cortex, we'll select EEG channels that capture activity from this region. Key channels include:
- C3: Located over the left motor cortex, sensitive to right-hand motor imagery.
- C4: Located over the right motor cortex, sensitive to left-hand motor imagery.
- Cz: Located over the midline, often used as a reference or to capture general motor activity.
# Select the desired channels
channels = ['C3', 'C4', 'Cz']
# Create a new raw object with only the selected channels
raw_selected = raw.pick_channels(channels)
Filtering: Isolating Mu and Beta Rhythms
We'll apply a band-pass filter to isolate the mu (8-12 Hz) and beta (13-30 Hz) frequency bands, as these rhythms exhibit the most prominent ERD/ERS patterns during motor imagery.
# Apply a band-pass filter from 8 Hz to 30 Hz
raw_filtered = raw_selected.filter(l_freq=8, h_freq=30)
This filtering step removes irrelevant frequencies and enhances the signal-to-noise ratio for detecting motor imagery-related brain activity.
Artifact Removal: Enhancing Data Quality (Optional)
Depending on the dataset and the quality of the recordings, we might need to apply artifact removal techniques. Independent Component Analysis (ICA) is particularly useful for identifying and removing artifacts like eye blinks, muscle activity, and heartbeats, which can contaminate our motor imagery signals. MNE-Python provides functions for performing ICA and visualizing the components, allowing us to select and remove those associated with artifacts. This step can significantly improve the accuracy and reliability of our motor imagery BCI.
3. Epoching and Visualizing: Zooming in on Motor Imagery
Now that we've preprocessed our EEG data, let's create epochs around the motor imagery cues, allowing us to focus on the brain activity specifically related to those imagined movements.
Defining Epochs: Capturing the Mental Rehearsal
The BCI Competition IV dataset 2a includes event markers indicating the onset of the motor imagery cues. We'll use these markers to create epochs, typically spanning a time window from a second before the cue to several seconds after it. This window captures the ERD and ERS patterns associated with motor imagery.
# Define event IDs for left and right hand motor imagery (refer to dataset documentation)
event_id = {'left_hand': 1, 'right_hand': 2}
# Set the epoch time window
tmin = -1 # 1 second before the cue
tmax = 4 # 4 seconds after the cue
# Create epochs
epochs = mne.Epochs(raw_filtered, events, event_id, tmin, tmax, baseline=(-1, 0), preload=True)
Baseline Correction: Removing Pre-Imagery Bias
We'll apply baseline correction to remove any pre-existing bias in the EEG signal, ensuring that our analysis focuses on the changes specifically related to motor imagery.
Visualizing: Inspecting and Gaining Insights
- Plotting Epochs: Use epochs.plot() to visualize individual epochs, inspecting for artifacts and observing the general patterns of brain activity during motor imagery.
- Topographical Maps: Use epochs['left_hand'].average().plot_topomap() and epochs['right_hand'].average().plot_topomap() to visualize the scalp distribution of mu and beta power changes during left and right hand motor imagery. These maps can help validate our channel selection and confirm that the ERD patterns are localized over the expected motor cortex areas.
4. Feature Extraction with Common Spatial Patterns (CSP): Maximizing Class Differences
Common Spatial Patterns (CSP) is a spatial filtering technique specifically designed to extract features that best discriminate between two classes of EEG data. In our case, these classes are left-hand and right-hand motor imagery.
Understanding CSP: Finding Optimal Spatial Filters
CSP seeks to find spatial filters that maximize the variance of one class while minimizing the variance of the other. It achieves this by solving an eigenvalue problem based on the covariance matrices of the two classes. The resulting spatial filters project the EEG data onto a new space where the classes are more easily separable
.
Applying CSP: MNE-Python's CSP Function
MNE-Python's mne.decoding.CSP() function makes it easy to extract CSP features:
from mne.decoding import CSP
# Create a CSP object
csp = CSP(n_components=4, reg=None, log=True, norm_trace=False)
# Fit the CSP to the epochs data
csp.fit(epochs['left_hand'].get_data(), epochs['right_hand'].get_data())
# Transform the epochs data using the CSP filters
X_csp = csp.transform(epochs.get_data())
Interpreting CSP Filters: Mapping Brain Activity
The CSP spatial filters represent patterns of brain activity that differentiate between left and right hand motor imagery. By visualizing these filters, we can gain insights into the underlying neural sources involved in these imagined movements.
Selecting CSP Components: Balancing Performance and Complexity
The n_components parameter in the CSP() function determines the number of CSP components to extract. Choosing the optimal number of components is crucial for balancing classification performance and model complexity. Too few components might not capture enough information, while too many can lead to overfitting. Cross-validation can help us find the optimal balance.
5. Classification with a Linear SVM: Decoding Motor Imagery
Choosing the Classifier: Linear SVM for Simplicity and Efficiency
We'll use a linear Support Vector Machine (SVM) to classify our motor imagery data. Linear SVMs are well-suited for this task due to their simplicity, efficiency, and ability to handle high-dimensional data. They seek to find a hyperplane that best separates the two classes in the feature space.
Training the Model: Learning from Spatial Patterns
from sklearn.svm import SVC
# Create a linear SVM classifier
svm = SVC(kernel='linear')
# Train the SVM model
svm.fit(X_csp_train, y_train)
Hyperparameter Tuning: Optimizing for Peak Performance
SVMs have hyperparameters, like the regularization parameter C, that control the model's complexity and generalization ability. Hyperparameter tuning, using techniques like grid search or cross-validation, helps us find the optimal values for these parameters to maximize classification accuracy.
Evaluating the Motor Imagery BCI: Measuring Mind Control
We've built our motor imagery BCI, but how well does it actually work? Evaluating its performance is crucial for understanding its capabilities and limitations, especially if we envision real-world applications.
Cross-Validation: Assessing Generalizability
To obtain a reliable estimate of our BCI's performance, we'll employ k-fold cross-validation. This technique helps us assess how well our model generalizes to unseen data, providing a more realistic measure of its real-world performance.
from sklearn.model_selection import cross_val_score
# Perform 5-fold cross-validation
scores = cross_val_score(svm, X_csp, y, cv=5)
# Print the average accuracy across the folds
print("Average accuracy: %0.2f" % scores.mean())
Performance Metrics: Beyond Simple Accuracy
- Accuracy: While accuracy, the proportion of correctly classified instances, is a useful starting point, it doesn't tell the whole story. For imbalanced datasets (where one class has significantly more samples than the other), accuracy can be misleading.
- Kappa Coefficient: The Kappa coefficient (κ) measures the agreement between the classifier's predictions and the true labels, taking into account the possibility of chance agreement. A Kappa value of 1 indicates perfect agreement, while 0 indicates agreement equivalent to chance. Kappa is a more robust metric than accuracy, especially for imbalanced datasets.
- Information Transfer Rate (ITR): ITR quantifies the amount of information transmitted by the BCI per unit of time, considering both accuracy and the number of possible choices. A higher ITR indicates a faster and more efficient communication system.
- Sensitivity and Specificity: These metrics provide a more nuanced view of classification performance. Sensitivity measures the proportion of correctly classified positive instances (e.g., correctly identifying left-hand imagery), while specificity measures the proportion of correctly classified negative instances (e.g., correctly identifying right-hand imagery).
Practical Implications: From Benchmarks to Real-World Use
Evaluating a motor imagery BCI goes beyond just looking at numbers. We need to consider the practical implications of its performance:
- Minimum Accuracy Requirements: Real-world applications often have minimum accuracy thresholds. For example, a neuroprosthetic controlled by a motor imagery BCI might require an accuracy of over 90% to ensure safe and reliable operation.
- User Experience: Beyond accuracy, factors like speed, ease of use, and mental effort also contribute to the overall user experience.
Unlocking the Potential of Motor Imagery BCIs
We've successfully built a basic motor imagery BCI, witnessing the power of EEG, signal processing, and machine learning to decode movement intentions directly from brain signals. Motor imagery BCIs hold immense potential for a wide range of applications, offering new possibilities for individuals with disabilities, stroke rehabilitation, and even immersive gaming experiences.
Resources for Further Reading
- Review article: EEG-Based Brain-Computer Interfaces Using Motor-Imagery: Techniques and Challenges https://www.ncbi.nlm.nih.gov/pmc/articles/PMC6471241/
- Review article: A review of critical challenges in MI-BCI: From conventional to deep learning methods https://www.sciencedirect.com/science/article/abs/pii/S016502702200262X
- BCI Competition IV Dataset 2a https://www.bbci.de/competition/iv/desc_2a.pdf
From Motor Imagery to Advanced BCI Paradigms
This concludes our exploration of building a motor imagery BCI. You've gained valuable insights into the neural basis of motor imagery, learned how to extract features using CSP, trained a classifier to decode movement intentions, and evaluated the performance of your BCI model.
In our final blog post, we'll explore the exciting frontier of advanced BCI paradigms and future directions. We'll delve into concepts like hybrid BCIs, adaptive algorithms, ethical considerations, and the ever-expanding possibilities that lie ahead in the world of brain-computer interfaces. Stay tuned for a glimpse into the future of mind-controlled technology!

Welcome back to our BCI crash course! We've explored the foundations of BCIs, delved into the intricacies of brain signals, mastered the art of signal processing, and learned how to train intelligent algorithms to decode those signals. Now, we are ready to put all this knowledge into action by building a real-world BCI application: a P300 speller. P300 spellers are a groundbreaking technology that allows individuals with severe motor impairments to communicate by simply focusing their attention on letters on a screen. By harnessing the power of the P300 event-related potential, a brain response elicited by rare or surprising stimuli, these spellers open up a world of communication possibilities for those who might otherwise struggle to express themselves. In this blog, we will guide you through the step-by-step process of building a P300 speller using Python, MNE-Python, and scikit-learn. Get ready for a hands-on adventure in BCI development as we translate brainwaves into letters and words!
Step-by-Step Implementation: A Hands-on BCI Project
1. Loading the Dataset
Introducing the BNCI Horizon 2020 Dataset: A Rich Resource for P300 Speller Development
For this project, we'll use the BNCI Horizon 2020 dataset, a publicly available EEG dataset specifically designed for P300 speller research. This dataset offers several advantages:
- Large Sample Size: It includes recordings from a substantial number of participants, providing a diverse range of P300 responses.
- Standardized Paradigm: The dataset follows a standardized experimental protocol, ensuring consistency and comparability across recordings.
- Detailed Metadata: It provides comprehensive metadata, including information about stimulus presentation, participant responses, and electrode locations.
This dataset is well-suited for our P300 speller project because it provides high-quality EEG data recorded during a classic P300 speller paradigm, allowing us to focus on the core signal processing and machine learning steps involved in building a functional speller.
Loading the Data with MNE-Python: Accessing the Brainwave Symphony
To load the BNCI Horizon 2020 dataset using MNE-Python, you'll need to download the data files from the dataset's website (http://bnci-horizon-2020.eu/database/data-sets). Once you have the files, you can use the following code snippet to load a specific participant's data:
import mne
# Set the path to the dataset directory
data_path = '<path_to_dataset_directory>'
# Load the raw EEG data for a specific participant
raw = mne.io.read_raw_gdf(data_path + '/A01T.gdf', preload=True)
Replace <path_to_dataset_directory> with the actual path to the directory where you've stored the dataset files. This code loads the EEG data for participant "A01" during the training session ("T").
2. Data Preprocessing: Refining the EEG Signals for P300 Detection
Raw EEG data is often a mixture of brain signals, artifacts, and noise. Before we can effectively detect the P300 component, we need to clean up the data and isolate the relevant frequencies.
Channel Selection: Focusing on the P300's Neighborhood
The P300 component is typically most prominent over the central-parietal region of the scalp. Therefore, we'll select channels that capture activity from this area. Commonly used channels for P300 detection include:
- Cz: The electrode located at the vertex of the head, directly over the central sulcus.
- Pz: The electrode located over the parietal lobe, slightly posterior to Cz.
- Surrounding Electrodes: Additional electrodes surrounding Cz and Pz, such as CPz, FCz, and P3/P4, can also provide valuable information.
These electrodes are chosen because they tend to be most sensitive to the positive voltage deflection that characterizes the P300 response.
# Select the desired channels
channels = ['Cz', 'Pz', 'CPz', 'FCz', 'P3', 'P4']
# Create a new raw object with only the selected channels
raw_selected = raw.pick_channels(channels)
Filtering: Tuning into the P300 Frequency
The P300 component is a relatively slow brainwave, typically occurring in the frequency range of 0.1 Hz to 10 Hz. Filtering helps us remove unwanted frequencies outside this range, enhancing the signal-to-noise ratio for P300 detection.
We'll apply a band-pass filter to the selected EEG channels, using cutoff frequencies of 0.1 Hz and 10 Hz:
# Apply a band-pass filter from 0.1 Hz to 10 Hz
raw_filtered = raw_selected.filter(l_freq=0.1, h_freq=10)
This filter removes slow drifts (below 0.1 Hz) and high-frequency noise (above 10 Hz), allowing the P300 component to stand out more clearly.
Artifact Removal (Optional): Combating Unwanted Signals
Depending on the quality of the EEG data and the presence of artifacts, we might need to apply additional artifact removal techniques. Independent Component Analysis (ICA) is a powerful method for separating independent sources of activity in EEG recordings. If the BNCI Horizon 2020 dataset contains significant artifacts, we can use ICA to identify and remove components related to eye blinks, muscle activity, or other sources of interference.
3. Epoching and Averaging: Isolating the P300 Response
To capture the brain's response to specific stimuli, we'll create epochs, time-locked segments of EEG data centered around events of interest.
Defining Epochs: Capturing the P300 Time Window
We'll define epochs around both target stimuli (the letters the user is focusing on) and non-target stimuli (all other letters). The epoch time window should capture the P300 response, typically occurring between 300 and 500 milliseconds after the stimulus onset. We'll use a window of -200 ms to 800 ms to include a baseline period and capture the full P300 waveform.
# Define event IDs for target and non-target stimuli (refer to dataset documentation)
event_id = {'target': 1, 'non-target': 0}
# Set the epoch time window
tmin = -0.2 # 200 ms before stimulus onset
tmax = 0.8 # 800 ms after stimulus onset
# Create epochs
epochs = mne.Epochs(raw_filtered, events, event_id, tmin, tmax, baseline=(-0.2, 0), preload=True)
Baseline Correction: Removing Pre-Stimulus Bias
Baseline correction involves subtracting the average activity during the baseline period (-200 ms to 0 ms) from each epoch. This removes any pre-existing bias in the EEG signal, ensuring that the measured response is truly due to the stimulus.
Averaging Evoked Responses: Enhancing the P300 Signal
To enhance the P300 signal and reduce random noise, we'll average the epochs for target and non-target stimuli separately. This averaging process reveals the event-related potential (ERP), a characteristic waveform reflecting the brain's response to the stimulus.
# Average the epochs for target and non-target stimuli
evoked_target = epochs['target'].average()
evoked_non_target = epochs['non-target'].average()
4. Feature Extraction: Quantifying the P300
Selecting Features: Capturing the P300's Signature
The P300 component is characterized by a positive voltage deflection peaking around 300-500 ms after the stimulus onset. We'll select features that capture this signature:
- Peak Amplitude: The maximum amplitude of the P300 component.
- Mean Amplitude: The average amplitude within a specific time window around the P300 peak.
- Latency: The time it takes for the P300 component to reach its peak amplitude.
These features provide a quantitative representation of the P300 response, allowing us to train a classifier to distinguish between target and non-target stimuli.
Extracting Features: From Waveforms to Numbers
We can extract these features from the averaged evoked responses using MNE-Python's functions:
# Extract peak amplitude
peak_amplitude_target = evoked_target.get_data().max(axis=1)
peak_amplitude_non_target = evoked_non_target.get_data().max(axis=1)
# Extract mean amplitude within a time window (e.g., 300 ms to 500 ms)
mean_amplitude_target = evoked_target.crop(tmin=0.3, tmax=0.5).get_data().mean(axis=1)
mean_amplitude_non_target = evoked_non_target.crop(tmin=0.3, tmax=0.5).get_data().mean(axis=1)
# Extract latency of the P300 peak
latency_target = evoked_target.get_peak(tmin=0.3, tmax=0.5)[1]
latency_non_target = evoked_non_target.get_peak(tmin=0.3, tmax=0.5)[1]
5. Classification: Training the Brainwave Decoder
Choosing a Classifier: LDA for P300 Speller Decoding
Linear Discriminant Analysis (LDA) is a suitable classifier for P300 spellers due to its simplicity, efficiency, and ability to handle high-dimensional data. It seeks to find a linear combination of features that best separates the classes (target vs. non-target).
Training the Model: Learning from Brainwaves
We'll train the LDA classifier using the extracted features:
from sklearn.discriminant_analysis import LinearDiscriminantAnalysis
# Create an LDA object
lda = LinearDiscriminantAnalysis()
# Combine the features into a data matrix
X = np.vstack((peak_amplitude_target, peak_amplitude_non_target,
mean_amplitude_target, mean_amplitude_non_target,
latency_target, latency_non_target)).T
# Create a label vector (1 for target, 0 for non-target)
y = np.concatenate((np.ones(len(peak_amplitude_target)), np.zeros(len(peak_amplitude_non_target))))
# Train the LDA model
lda.fit(X, y)
Feature selection plays a crucial role here. By choosing features that effectively capture the P300 response, we improve the classifier's ability to distinguish between target and non-target stimuli.
6. Visualization: Validating Our Progress
Visualizing Preprocessed Data and P300 Responses
Visualizations help us understand the data and validate our preprocessing steps:
- Plot Averaged Epochs: Use evoked_target.plot() and evoked_non_target.plot() to visualize the average target and non-target epochs, confirming the presence of the P300 component in the target epochs.
- Topographical Plot: Use evoked_target.plot_topomap() to visualize the scalp distribution of the P300 component, ensuring it's most prominent over the expected central-parietal region.
Performance Evaluation: Assessing Speller Accuracy
Now that we've built our P300 speller, it's crucial to evaluate its performance. We need to assess how accurately it can distinguish between target and non-target stimuli, and consider practical factors that might influence its usability in real-world settings.
Cross-Validation: Ensuring Robustness and Generalizability
To obtain a reliable estimate of our speller's performance, we'll use k-fold cross-validation. This technique involves splitting the data into k folds, training the model on k-1 folds, and testing it on the remaining fold. Repeating this process k times, with each fold serving as the test set once, gives us a robust measure of the model's ability to generalize to unseen data.
from sklearn.model_selection import cross_val_score
# Perform 5-fold cross-validation
scores = cross_val_score(lda, X, y, cv=5)
# Print the average accuracy across the folds
print("Average accuracy: %0.2f" % scores.mean())
This code performs 5-fold cross-validation using our trained LDA classifier and prints the average accuracy across the folds.
Metrics for P300 Spellers: Beyond Accuracy
While accuracy is a key metric for P300 spellers, indicating the proportion of correctly classified stimuli, other metrics provide additional insights:
- Information Transfer Rate (ITR): Measures the speed of communication, taking into account the number of possible choices and the accuracy of selection. A higher ITR indicates a faster and more efficient speller.
Practical Considerations: Bridging the Gap to Real-World Use
Several practical factors can influence the performance and usability of P300 spellers:
- User Variability: P300 responses can vary significantly between individuals due to factors like age, attention, and neurological conditions. To address this, personalized calibration is crucial, where the speller is adjusted to each user's unique brain responses. Adaptive algorithms can also be employed to continuously adjust the speller based on the user's performance.
- Fatigue and Attention: Prolonged use can lead to fatigue and decreased attention, affecting P300 responses and speller accuracy. Strategies to mitigate this include incorporating breaks, using engaging stimuli, and employing algorithms that can detect and adapt to changes in user state.
- Training Duration: The amount of training a user receives can impact their proficiency with the speller. Sufficient training is essential for users to learn to control their P300 responses and achieve optimal performance.
Empowering Communication with P300 Spellers
We've successfully built a P300 speller, witnessing firsthand the power of EEG, signal processing, and machine learning to create a functional BCI application. These spellers hold immense potential as a communication tool, enabling individuals with severe motor impairments to express themselves, connect with others, and participate more fully in the world.
Further Reading and Resources
- Review article: Pan J et al. Advances in P300 brain-computer interface spellers: toward paradigm design and performance evaluation. Front Hum Neurosci. 2022 Dec 21;16:1077717. doi: 10.3389/fnhum.2022.1077717. PMID: 36618996; PMCID: PMC9810759.
- Dataset: BNCI Horizon 2020 P300 dataset: http://bnci-horizon-2020.eu/database/data-sets
- Tutorial: PyQt documentation for GUI development (optional): https://doc.qt.io/qtforpython/
Future Directions: Advancing P300 Speller Technology
The field of P300 speller development is constantly evolving. Emerging trends include:
- Deep Learning: Applying deep learning algorithms to improve P300 detection accuracy and robustness.
- Multimodal BCIs: Combining EEG with other brain imaging modalities (e.g., fNIRS) or physiological signals (e.g., eye tracking) to enhance speller performance.
- Hybrid Approaches: Integrating P300 spellers with other BCI paradigms (e.g., motor imagery) to create more flexible and versatile communication systems.
Next Stop: Motor Imagery BCIs
In the next blog post, we'll explore motor imagery BCIs, a fascinating paradigm where users control devices by simply imagining movements. We'll dive into the brain signals associated with motor imagery, learn how to extract features, and build a classifier to decode these intentions.